Peter Voss (How and when will we build really intelligent machines?)
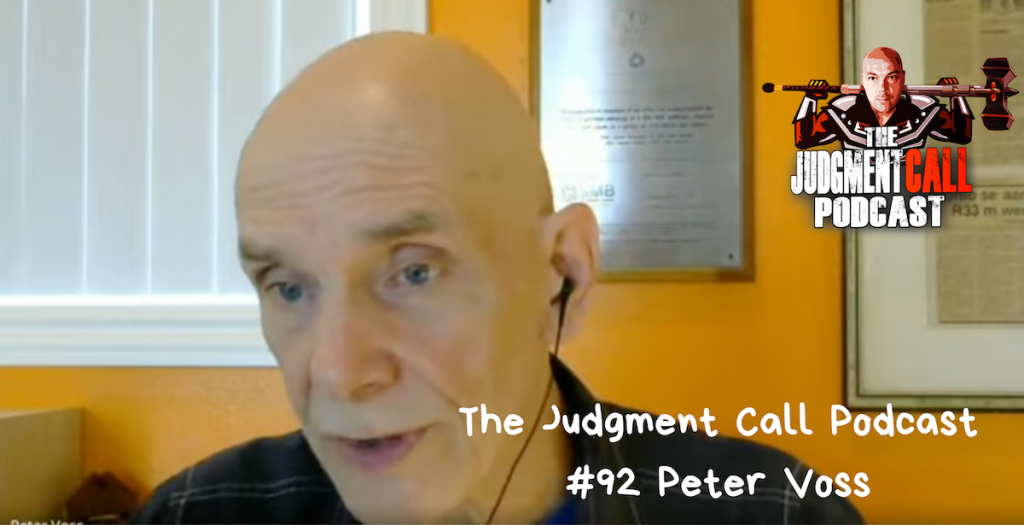
- 00:00:30 How did Peter get started studying intelligence and how he envisions computers and AI should become more intelligent?
- 00:06:31 What better approach can we take to build a thinking machine?
- 00:12:18 When will we have machines that can build and improve themselves?
- 00:16:50 How will the third wave of AI look like?
- 00:19:06 Is GPT-3 like magic or is it a failure?
- 00:21:06 Will we need to create a ‘Free Will’ to create an intelligence machine?
- 00:34:10 How self awareness and abstract concepts are a necessary predecessor to real intelligence?
- 00:39:49 Is overly logical thinking a hindrance in modelling ‘social functions’ in computers?
- 00:45:41 Can ‘real intelligence’ be an emergent property of ‘dumber algorithms’?
- 00:49:09 How aigo.ai is creating a chatbot with a brain?
- 00:59:03 How does aigo.ai learn from customer interactions?
- 01:12:15 Will we all live the lifes of kings soon? What abundance can we expect from the Singularity?
- 01:21:15 How much money should be allocated ‘Third Wave AI’?
You may watch this episode on Youtube – # 92 Peter Voss (How and when will we build really intelligent machines?).
Peter Voss is a serial entrepreneur and currently runs aigo.ai. The company implements a ‘Third Wave of AI’ with deep understanding of natural language, have short- and long-term memory, learn interactively, use context, and are able to reason and hold meaningful ongoing conversations.
Big Thanks to our Sponsors!
ExpressVPN – Claim back your Internet privacy for less than $10 a month!
Mighty Travels Premium – incredible airfare and hotel deals – so everyone can afford to fly Business Class and book 5 Star Hotels! Sign up for free!
Divvy – get business credit without a personal guarantee and 21st century spend management plus earn 7x rewards on restaurants & more. Get started for free!
Brex – get a business account, a credit card, spend management & convertible rewards for every dollar you spend. Plus now earn $250 just for signing up (Terms & Conditions apply).
Peter, thanks a lot for coming into the George Macal podcast, we really appreciate it. Yeah, thanks for having me. Hey, absolutely. You know, you are, you spend a lot of time with artificial intelligence from what I read, you actually work on your second company now monetizing research that you’ve done over the years of artificial intelligence. You can help us understand that a little bit better and what is kind of your secret sauce? What are you specializing in? Yes, so there’s a little bit of history here. I started as an electronics engineer, so, you know, really understanding electronics and computers from the hardware side. Then I fell in love with software and my electronics company turned into a software company developed an ERP software system. That company became very successful. We grew very rapidly and actually did an IPO. So that was great. But when I exited that company, I had time to really think about, you know, what big project do I want to tackle next? And the thing that struck me is that software is really dumb, you know, and I’m very proud of my own software, but still, it doesn’t have any common sense. It doesn’t learn. It doesn’t reason and so on. So that’s really what struck me started on my journey of artificial intelligence to figure out how we can build intelligence software. So I actually took of five years to study different aspects of intelligence to really deeply understand what is intelligence? What are we looking for in AI? And you know, I started at philosophy, epistemology, theory of knowledge. How do we know anything? What is reality? You know? Sounds like a deep dive you took there. Yeah. Right. How do we know anything? Absolutely. And, you know, what do IQ tests measure? So I went into psychometrics and cognitive psychology, understanding how do children learn, you know, what experimentation had been done on animal intelligence and how that differs from our intelligence. And generally doing research on what work had been done in AI over the decades. And during that journey, I, you know, got a much better understanding of what we’re looking for in intelligence. And that brought me to a point where it was rather odd looking at what people were doing in the field of AI compared to, you know, the original vision of artificial intelligence when the term was coined some 60 plus years ago. People really wanted to build a thinking machine, a machine that can think and learn and reason the way humans do. And they thought they could do this, you know, like in a few years they would achieve that. Now, of course, it turned out to be much, much harder. So over the decades, what happened with AI is it moved away from this initial vision and ideal and goal to narrow AI. So basically people started saying, hey, if we can just take one particular problem that humans can solve, and we can automate that, then we’ve got AI. And you know, deep blue is a perfect example of that, you know, the world chess champion that IBM built this, you know, very powerful chess playing machine. But that’s narrow AI. And over the years, what the conclusion I came to is it’s really very, very different because what you’re doing is you’re taking a problem and you’re using human intelligence to figure out how to use a computer to solve the problem. And then you write the program with really the human solution. And it’s the intelligence actually resides in the designer of the program and not in the program itself. So we really lost our way on AI over the decades that it’s become narrow AI. Yeah, people feel very, very proud about the progress that we’ve made in the last couple of, I’d say, 10 years, where we finally have a relatively abstract way to solve narrow AI problems. You kind of dimension them a little bit, so that’s actually not what we set out in the 60s, right? And artificial intelligence first came about as a term. So it’s something that you literally just download from GitHub, prepare a data set, let it run, and it spits out an answer. And that’s pretty amazing, right? There’s this example from, I think it’s the lane changing auto 114, self driving cars, which was 10, 15 years ago, something that would take 20 engineers and a lot of research and it still had a pretty big error rate. And now you download it from GitHub and it works right away. You just connect to the proper images. So the progress that we have with this world of the generic model in narrow AI seems to be pretty stunning. And I think what the idea is to now is that we eventually have enough of these building blocks that we can put together the 1960s version of the thinking computer, right? I think this is at least the hope right now, and when we talk about the singularity, something that we’re going to see in the next 20 years, do you feel like we have to redesign what we see so far and we need a different approach to get to this next level of AI? Yes, I absolutely do. And it’s obviously not a simple topic and quite controversial because literally trillions of dollars are at play with deep learning, machine learning, the specialized hardware that’s being designed. I mean, they’re multi, multi billion dollar companies riding on the success of deep learning, machine learning. But in my view, this is not artificial intelligence in the form that was envisaged at all. It’s a very, very powerful tool that can be used. But the intelligence still resides essentially in the data scientist. I mean, it’s non trivial, you know, people make it sound, well, we just build this model and it can do things. But if you actually start working with these systems, it’s non trivial to decide what data inputs to have to miss out to the data and to build, you know, figure out what kind of architecture and what model will actually work. Now, of course, there are automated systems that might iterate through hundreds or even thousands of different models and try them. So but it’s a dumb process. It’s basically, you know, by trial and error. But let me go back a little bit and talk about what I think we need or why we need to do something fundamentally different to achieve that original vision of having a thinking machine. So the conclusion of the research in 2001, I then started my first AI company initially in research phase, had about 10 people that are hired and we basically took the ideas that I had and started experimenting around with them. At that same time, 2001, myself and two other people, we actually coined the term artificial general intelligence or AGI and wrote a book on the topic. And that was really to say, we want to get back to the roots of AI to have machines that can think and learn and reason. And just to jump ahead a little bit now, close the circle. Keep learning, machine learning, have some severe limitations or let me talk about what intelligence requires. So if you interact with a person and you regard them as intelligent, you expect them to have certain characteristics. You expect them to be able to learn or what an AI is called one shot learning. If they tell you something, you expect that, if you tell them something, you expect that person to remember it and to use that knowledge immediately, not to need thousands of examples. If you have a child, you can show them one picture of a giraffe and they’ll be able to recognize giraffes, toy giraffes, pink giraffes or whatever or an elephant or whatever. So this one shot learning is one thing you expect a system to be able to do. You expect them to have deep understanding of what you’re saying. So if there’s some kind of an input you get that the implications are clear to that person. Why are you saying it? Theory of mind, what knowledge do you have? What is gone before? So reasoning is involved, one shot learning is involved. And these statistical systems that work with huge amounts of input data basically just take a snapshot of all the input data and create a model from that. But that model is essentially a read only model. It doesn’t learn, it doesn’t reason. And that’s inherent in the approach of deep learning, machine learning. Yeah, certainly just what approach we feel like. I think everyone was surprised how well it works in certain areas. And so it’s really taking off, and we’ve seen this from the search engines especially. I think we’ve seen this with Google and Facebook, they sit on this massive trove of data. They didn’t really know what to do with it. And so deep learning really fit into this paradigm that they use all this data and try to extract some knowledge from it. Now the real world doesn’t have such well designed data sets. Data is all over the place and it has different preconditions to it that we don’t really see in the data. And now that’s different on Facebook and Google, and I think this is where most of the innovation was the last 10 years. What I really like is how you say, well, the problem is actually that a lot of these preconditions have been set in motion by the designer. So the designer is the only intelligent part. And I see this when we think about ourselves. So when we are born with one gigabyte of data, this is what the DNA is. But all these really difficult models, how we learn how to see the world in a year or two years old, how we recognize a cat, how we recognize a giraffe, as you just said, with very few examples. We don’t come with tons of gigabytes and gigabytes of terabytes, petabytes of data. We come with very small instructions on how to build these models, how our brain eventually figures out how to build these models. So someone must have created this evolution of the designer. And that’s maybe where the real intelligence is. Maybe we see this with machines, the obviously the challenges. Once they figure out a working model, it’s immediately being distributed to all the machines that are connected through the internet. The fear is currently that a lot of people feel, well, once a machine, we get to this point that they can either design themselves or we help them with more advanced models. But they immediately, once they figure something out, every single machine on the planet has that model. So they will escape. They have to escape velocity to an intelligence, even if we start with more design and it looks really clumsy right now, they have to escape velocity that can quickly bring them off this planet. And there’s very little for us we can do. We can’t follow them. Yeah. I mean, the intelligence explosion of when a machine becomes smart enough to capable enough to redesign itself and improve itself, I think I believe that is a very realistic scenario. So I do believe that will happen. I’m not sure what the theoretical maximum of intelligence is, you know, how intelligent can a system become before it becomes sort of self defeating in a way. And what I mean by that is you couldn’t just have a computer that gets bigger and bigger and bigger and become smarter and smarter because of course you have the speed of light, you have, you know, physical limitations of what it can do. And you have a combinatorial explosion of, you know, of scenarios you want to analyze. So there’s probably an optimum size of intelligence in a single unit. Then you need, you know, cooperation between different units. They say the total limit of energy available in the universe is an absolute limit and it’s not that far out, right? I mean, we can count all the stars and we have the amount of energy available. Right. But I mean, at the moment where we are right now, I mean, yes, one can talk about this sort of future and how crazy that is when you have computers that can redesign, design themselves and improve their own design quicker than humans can and whatever the physical limitations on actually building the hardware and so on. But right now we are such a long way away from this. I mean, when you look at, as you know, as you say, it’s just shocking how well these deep learning machine learning systems actually function if you give them the right amount of data, you know, what you can achieve. So speech recognition has, you know, improved tremendously, image recognition has improved tremendously and unfortunately targeted advertising, you know, which is really driving the deep learning machine learning train, you know, that has, you know, improved a lot. You mentioned that, you know, Google search has improved. I don’t really see that so much. I mean, before deep learning go back 10 years, we didn’t have deep learning machine learning. I don’t really see that my Google searches are, you know, have any more understanding of what I’m looking for. So I don’t mind. I don’t know. This is the customization. I compare it with being from time to time. So two browsers and I do like a specific comparisons. And I feel it’s, if it’s just pure luck, but I’d say 90% of the time, it’s exactly what I wanted and that’s, you know, well, these are not necessarily the main sites, 20 different sites on the internet. I get most of the traffic. I’m very happy with Google. I don’t know if this personalization feature or that’s something that they’ve just other because they have bigger click click data than anyone else, it’s, it’s far away from me and that long tail compared to me. Yeah. Yes. I mean, it is by far the most powerful search engine. I, I mean, I’m not actually familiar with how much deep learning machine learning they use in their search now, whether that has improved their search, maybe it has, I, I don’t know. But I think fundamentally deep learning machine learning or statistical systems are not going to get us to, to true intelligence because they lack these fundamental requirements of intelligence, of being able to learn interactively to use context and to be able to reason. And you know, and that’s sort of inherent in the approach. I mean, people, you know, there’s some research being done where they’re trying to get one shot learning working in, in, in these networks and that, but it’s really the data representation and knowledge representation is all wrong. It’s a black box. You can’t. Yeah. I mean, they’re just a huge amount of limitations. And I think one of the models that I find quite useful is when DARPA presented, gave a presentation a few years ago, where they spoke about the third wave of AI and you mentioned it. Yeah. The three waves of AI. And I think that to me seems, seems to really capture what, what I think the problem is. So the first wave is basically, you know, logic based systems that, that were prominent in the 70s, 80s and 90s, expert systems, but you know, really like psych would fit into that mainly logic, logic, logic based systems. And then of course we’ve now had this revolution of machine learning, deep learning where they finally figured out how they could use neural networks and make them work, you know, whereas over the decade, they couldn’t really get neural networks to do too many useful things. And then about 10 years ago, we had this breakthrough when companies with a lot of data, a lot of computing power could, you know, suddenly make, make these things work really, really well in, in certain applications. Well, one, one thing, and I think this fits into this breakthrough would be when we look at GPT three, which isn’t a crazy difficult model, right, it took about 20 million to, to actually run. And it’s, it’s something that’s a relatively small team, like it’s not exactly a Manhattan project. Let’s put it this way, right? So it’s, it’s a limited team. And it took basically data that’s available from, from the web. So it’s relatively public data. It’s not super secret. What happened to, is that it came up with a lot of, and I have, I have this, you can need a developer ID to use it, so I’ve been playing with it for the last couple of weeks and it’s been out since July. It doesn’t know what it’s doing, right? But it’s a bit, it’s, it’s throwing darts, but it’s throwing darts in a matter that you feel like, whoa, that’s really good, right? It writes HTML code, it writes some, some other codes it, it can create essays. It’s kind of on the level I’d say we’re early stage teenagers. Now, teenagers grant it, sometimes they have understanding on what they’re doing, often they don’t, because they don’t care, right? But it’s, it’s, if a 12 year old’s writing essay or an 11 year old’s, it kind of looks like open AI. And sometimes open AI advances beyond that stage. So I feel, even if it’s accidental and even if it’s pure statistic, which is, which it is, it doesn’t really know what’s going on, the results are pretty stunning. I would disagree. I, I think they, it’s, they really like parlor tricks and, and they know we’re near 12 year old in terms of learning or understanding or, or, or anything like that. And I think it’s fundamentally the wrong architecture. I mean, what we’re seeing now is if you throw 10 times as much data at it and computing power, you know, if you use the electricity of Manhattan, you know, to, to build these new, new models, the improvements are becoming smaller and smaller and the thing is there’s still no, no learning, no reasoning, no understanding. If you try to, yes, they look impressive, you know, because the patterns that it outputs are obviously sophisticated patterns that, that humans have created, you know, what, what the model was built on. But you try to have, you try to use, I mean, one of the tests would be to try and use it commercially and, you know, where you can’t just have random babbling that impresses you, you know, that, oh, wow, this, this, you know, this could be poetry or it could be a rock song or it could be a badly written essay by something, you know, it doesn’t make any sense. Well, as soon as you want something that actually makes sense and that you can rely on the system is completely lost. You, you know, it’s 99% fits, it’s correct. So they, they did this study, I think it’s a white paper came out a couple of weeks ago using OpenAI, they did the summaries of other white papers or longer text, so even podcasts. So this is a TLDR on Reddit and what the machine did was way better in, in that study, I wouldn’t say way better, but it was slightly better than what human editors would do. And that was pretty exciting. And it was rated by other humans, right? So the humans in the end rated what was actually the better summary and it came out at OpenAI, writes better summaries than other crowdsourced, good editors on Reddit. And that doesn’t mean it works 100%, it’s like this 1%, 2% where it’s complete garbage and it has no idea what’s going on and it doesn’t have any idea of the other 99%, but it’s still pretty cool for, and as you said earlier, then it’s still a kind of a sense of a narrow AI. The question obviously is, how do we scale up and how we, how do we generate this understanding? How do we get to this, this, maybe it’s a bit of a question of a free will, where you realize that there is a time horizon, there is something that I want, me as a consciousness, right? I want something, how do I get there, how do I define these goals, there’s lots of things involved, but how do I actually make sense of the world around me? How do we get there? Is there something you’re working on right now, is there ones out there that can do this? So to complete going back to DARPA’s third wave, obviously, we’ve spoken about the first wave and the second wave, so I believe that there’s some importance in the third wave. So when DARPA talk about the second wave being statistical systems, and no, I do not believe that there is a direct path, it doesn’t matter how much processing power, how much data you throw at it, you’re not going to get this real intelligence, yes, you can cherry pick certain applications where the system is good, but we’ve heard claims of that natural language understanding is better than humans or 99% or something, it’s complete garbage, there is no understanding, and you can, if you have the right tests, you can test that very quickly and tease it out, but talking about the third wave, so what is the third wave? The third wave is basically inherently systems that are designed from the ground up to have the components required for intelligence, so that they have interactive learning, they have deep understanding, they have the ability to reason, and they have common sense. So what do you need to do that? Now each of these terms are obviously quite complex to unpack. Now the third wave, the way I see it is, it’s essentially a cognitive architecture. So you say, well, we have to represent data in a certain way, that is not just a black box, or that is scrutable, that the system can explain why it’s doing things, it can reason about things, and it can learn interactively, so I think that’s what you need, it’s fundamentally a different architecture, it’s very tempting to have this sort of silver bullet, to say you have this small algorithm, few hundred lines of code, and that’s all you need, and then you just throw a lot of data in computing power and it will magically create human level intelligence, but I don’t, I think the evidence is that that’s not the path to human level generally. We just haven’t found it yet, Peter, we just haven’t found it yet, well because it’s quite stunning, and I read your essay about free will, you, that you put out a couple of years ago, and it’s stunning, but I think this is very much related to me, and correct me if you see it differently, but free will is this element that we instinctively believe in, right, we as humans always think we have free will, we make the decision we are in control, but there’s obviously a lot of problems with that, A is if you give a certain kind of information to the subject, it will change their opinion, almost guaranteed, I mean there’s certain edge cases, but if you have the opportunity to present certain kinds of maybe biased information for a certain amount of time, six months, undisturbed, someone will change their mind, or pretty much anything, even their core beliefs of their individuality. The other problem is there’s so much stuff that streams into our unconsciousness, and only very few things actually make it to the consciousness, so there’s even more information that someone inside of us already makes a lot of decisions about if we should even worry about that, then there’s this whole other topic that you also write about is that the body and the nervous system, they predetermined a lot of our decisions, probably 99.9%. So then the question becomes, are we basically, are we already like a machine, that’s a carbon machine, that someone else may be designed, who had exactly the same problem, can be used this biological system that we are, that seems to be, we haven’t decoded it yet, or we have the DNA, but we don’t know how it actually translates itself into our organs, for instance, work, how our brain works, and how our consciousness works, do you feel that we learned this from biology, or we have to redesign and find it from scratch? Okay, a lot of different things here, so I think talk about free will on the one hand, and then on the other hand, what do we need to get to AI, if I sort of, the tail end of what you were talking about. So let me talk about the second one first, and we get back to free will. So the second one, I don’t believe that we need to look at biology in particular, to build AI, and sort of my favorite comparison there is, we’ve had flying machines for over 100 years, but we still know we’re near reverse engineering the bird. So it’s basically, learning something from nature, and what birds do, but that’s ultimately not what build the most effective flying machines, because as human engineers, we have very different strengths versus evolution, and we have very different materials that we’re working with, you know, on that, and the same is true for building thinking machines, the materials we’re working with are very different from what evolution had building our brains, and the, again, engineering us as engineers, as human engineers, very different from a blind process of evolution. So I think we can get inspiration from biology, and certainly how our brain or our mind works, but I don’t think that, you know, evolution or biology is the answer to get to AI. Going back to free will now, the topic of free will is actually really quite difficult, and usually sort of people go off on a track on free will, it’s something that obviously has strong, illicit, strong emotions in people, you know, if you say you don’t have free will or you do have free will and so on, but people usually don’t stop and really think what the definition is, what they actually mean by free will, and then often the flip side of free will is determinism, you know. So I think most discussions about free will are not really off the mark, missing the mark, because they don’t spend enough time explaining what they mean by free will and what their understanding of determinism, if that’s sort of the counter argument. I mean, if we’re talking about free will, that you could make decisions irrespective of evidence, irrespective of knowledge, irrespective of anything, like what’s the point? I mean, then it’s just like random, you know, random decisions, and that’s not what we mean by free will. I think the useful, we need to go back even one step and say, why do we even have this concept of free will, why is it an important concept in humans? And that gets us closer to the mark, because why it is an important concept is personal responsibility. If we didn’t have something, and let’s call it volition, you know, free will has such a lot of baggage, such an emotional, you could almost not use the word without triggering a whole lot of, you know, beliefs that go with it. If you say, why do we have something that we call free will or volition or whatever? Well, the reason this concept comes about, I think, is of personal responsibility. You know, are you as a person responsible for the decisions that you make? I think that’s really, and or to what degree are you responsible for that? But isn’t it just an illusion, isn’t this, we, our mind holds us responsible for it. So I agree with you. But isn’t that just in the end an illusion, because most of the data stream that we are exposed to, and all these things that happened before, we have no clue how they happened, why they happened, all the nature, all the entropy, we don’t see it, we don’t really interact with it. And we just keep up this idea of free will, which is really built into us, as you say, is an emotional need, always a craving that we have. But isn’t it enough that we have this illusion, it doesn’t matter if we have free will or not? Yeah. So you said you read one of my essay, free will essays. I actually wrote the first one where I argued that free will exists, and it’s compatible with determinism, so the compatibilist. And I found that about, you know, half the people I spoke to just couldn’t get on the same page, you know, they just basically said, no, there is no free will, and I couldn’t get anywhere. So I wrote the second essay a few years ago, where I say, we don’t have free will, but we have something better. So that was sort of, depending on what the perspective you take and what your interpretation of free will is, the explanation, I think the answer of it is, you could really start from both ends, you know, for one kind of explanation of free will, you can say, yes, we have free will. Or for another explanation, you can say, no, we don’t have free will, you know, fine. So we’re on the same page, we can agree. And now we can talk about what is it that makes us different from animals, or to what extent are we different from animals, if you can agree that animals are not responsible for their actions to the same extent. Like the narrow AI, right? You could say that animals put basically like a narrow AI, it depends on what animal we’re talking about. But let’s not use a mammal, let’s use a bird, maybe, right? So they have some specific solution and work for them. But from our understanding at least, there’s no language, there’s no civilization, obviously, and there’s no, they don’t have any understanding of the future. So for them, free will is not something they have to put resources in, because it makes no difference, right? You have to first figure out there is a future, and then you have to figure out, okay, what do I do about this future? And maybe some primates have some understanding about the future. I don’t know how much research you’ve done, but that’s about it, right? So that’s very few, and for me, that’s kind of a mystery, if consciousness and this understanding of the future is such a great tool made as the EPEX Predator, why didn’t anyone else at least go pretty far along to that travel that we’ve done? That’s kind of a mystery to me, because machines will have to go along the same track, right? They will go where the animal started and become human like. When you say, what is a mystery to you that nobody, what do you mean that no human has defined? Well, I find it a mystery that there’s only one specific part of the primates that developed all these advanced functions, and we feel they are pretty advanced, because at least they manifest themselves. We are changing the planet right now, so maybe we’re terraforming the planet. So it is definitely a big deal, and only very few primates, we are the descendants of it, and we can trace down our ancestors, literally one couple in Ethiopia. Only they developed this and nobody else, I find this quite strange. Well, I mean, you know, we are here as humans, that evolutionary path got us to where we have that understanding, we have that high level intelligence. And in fact, my studies of intelligence pretty much pinpointed what it is that makes us different from animals, and in brief, it’s our ability to form abstract concepts, and to form abstract concepts of abstract concepts, so that we have this sort of unlimited, you know, once you can form an abstract concept of another abstract concept, then you can go anywhere with that. And, you know, that’s the unique ability, but in evolution, clearly there was a trade off in terms of having that very flexible brain required a much longer nurturing period, you know, and obviously in the wild it’s difficult to survive, whereas if you pre programmed, you know, of what works and what doesn’t work, then that’s evolutionary much, much easier. So yes, we the first species that has developed this ability, and they seem suspicious to me, so suspicious, and for us it happened, I’d say 200,000 years ago, maybe 300,000, but it’s a long, relatively long time, you know, where you can say long or not long, but it seems to be decent enough that another species would have caught up to us, you know, that someone would have invented fire, the chimpanzees. It doesn’t seem to be such a big deal, but yet it’s not even in the cards right now. I find this really weird, maybe it’s just random, but it seems like we know that evolution works in two ways, right, or in many different ways, but one is you copy what works, so you see something there and then you learn it because it’s so much easier instead of reinventing the wheel, but none of the other primates could learn this from us. I find this really odd. I mean, that’s just a side story. Yeah, yeah, right. Okay. But it leads me to how do we replicate this, right? So if the animal is kind of replicated, so if it’s such a divine, unique thing, maybe divine is not the right word, and, you know, I don’t know if you remember 100 years ago, a lot of researchers, brain researchers said we can never build the brain because it’s just so complicated and it’s not going to happen and then, you know, now we have a lot of understanding about the brain, not exactly how it works, but we found out a lot of stuff. So I think we have to get, isn’t that something where we look at how animals work and how they don’t work, where we can figure out maybe where did this last step in evolution come from and how can we give this to machines, right? I think this is the step you’re talking about these days. Yes, exactly. And this is, you know, what I’ve pinpointed the difference is this ability to form abstract concepts. That really is the key and, you know, you need an AI to be able, right now we don’t have AI, the current crop of AI’s don’t really work conceptually. They don’t form concepts, never mind forming abstract concepts. It’s just not the way the direction of the research and the focus, you know, where all the money is right now, but you basically need to build an AI that can form concepts. And once you have that, it’s actually a relatively simple step from there to go, and I mean, from an evolutionary timescale to go from mammals to humans was a blink of an eye. It was actually, you know, very, very, very quick. It wasn’t billions or even millions of years. So the difference was basically to use that machinery that allows you to form first level concepts, to use that machinery and modify it that you can now form concepts of concepts. And you know, and then concepts of concepts of concepts. And that is really the unique ability that we have. And that is the key to high level or human level intelligence is the ability to think an abstract, to form abstract concepts and to think an abstract concept. Do we have a mathematical approach to this? Do we know how we can? And we know that math is basically an extremely abstract concept of reality. But do we have a mathematical set of function that describes it where we can say, well, this is the problem and we can, here’s a set of algebra and I can, this algebra, once I apply it, see my hypothesis works kind of like we discover physics, right? So we played around with the numbers long enough that we figured out the equations. Is that something that’s anywhere close to, to some extent, you know, when we come up with these equations, we don’t really know what we are after, right? So sometimes it’s a representation of what we see we want to prove. But sometimes these equations kind of like I’m having some of the more modern math in mind, we have the equation where we don’t really know what it means. We can prove they are right, but we don’t really know what that means for physics. Right. So no, I don’t think mathematics is the right tool. I mean, it’s obviously related to programming and necessary for programming, but it’s much more disciplined like organic chemistry, you know, for example, where you run out of steam with mathematics and, you know, you just have bits and pieces that you can put together, but the complexity is such that mathematical tools really aren’t, aren’t powerful enough for that. So I don’t think mathematics is the answer to solving the problem. In fact, this brings up quite an interesting point on my project. So in 2001, I started, you know, the first AI company to turn sort of the ideas that I came up with over my five year research into actual prototypes and, you know, experimented it with. We then came up with a cognitive engine that we could commercialize, so in my first company I commercialized it in the call center space. But the point I want to make here is I found that the people that are hired, I had some brilliant engineers over the years that I’ve hired, but some of them really couldn’t get on the right page as far as AI, the kind of AI that we want to have because they have a strong mathematical logic background and they try to shoehorn everything into, you know, strict logic, formal logic, mathematics or statistics. The people that I found could really help me make progress in building AI are people who are comfortable with cognitive psychology. So the reason for that is I think you first need to understand, have an understanding what intelligence is, you know, what learning is, how people learn, the sort of the, yeah, cognitive psychology, basically developmental psychology and understanding how the mind works, not the brain, how the mind works. I think that’s an important component and often cognitive psychologists aren’t good logicians or programmers and good programmers aren’t good cognitive psychologists. So I think you really need to have both aspects. You need to understand the problem from a cognitive psychology point of view, from understanding intelligence and what it entails. And then of course you need to have the technical knowledge to be able to build systems that can implement that. So no, I don’t think, again, it comes back to the sort of, we all love to have a magic silver bullet, you know, a formula, an algorithm, a mathematical model that we can say, oh, we’ve cracked AI, you know, we’ve got the code. Now all we have to do is, you know, run it on a computer, expose it to the world and hey presto, AI is solved. I don’t see that that’s going to happen at all. Yeah, it’s kind of this battle between the hard sciences, right, physics or STEM topics. And then on the other side, we have psychology and we have the humanities where we feel like, and I think it goes back and forth once we put it into an algorithm and that’s maybe the lack of the last 20 years and because some are conductors scale up so much in the last 20, 30 years, whatever we put into an algorithm, and I think this is where this love comes from, it can scale out for almost, there’s almost no cost involved, right, even if you can make something that even is so complicated now, nobody can run it like open AI, but in 10 years from now, you can run it on the iPhone. And I think this is where all of this excitement comes from, is that even if it’s crude and it’s very limited, but it’s very predictable where this will go, right, so we know that an iPhone in 10 years will have the computing power, not the intelligence, but way higher computing power than a million human brains, just core computing power, doesn’t mean it can do what the brain can do, but we can use this machine power to help us with guiding our decisions. It’s narrowing, right, this is very narrowing, I think. So I think that’s why all the engineers are drawn to this, because there’s so much from the hardware side, there’s such a huge push that comes every 12 months or 18 months. Technology doesn’t have that, right, so psychology, and it has the same topics people had a hundred years ago, and it has the same tools, and yes, it changes to a different psychometrics every couple of years, but in the end, they all seem very interchangeable. Yeah, of course, it’s very tempting to kind of throw money at deep learning, machine learning, because as you say, in some ways predictable, if we can have faster machines and bigger models and they’ll be better at image recognition or speech recognition or whatever, but we’re also seeing, as I mentioned earlier, that speech recognition over the last five years probably hasn’t improved that much, even though we have a hundred times bigger models. The technology is basically topping out of what it can do. Now of course, as you say, if you can have the power of the TPD3 model on your phone, there are certainly things that you can do that we can’t dream of doing today, but is it intelligence? Is it the kind of intelligence that we want that can actually solve general problems, human level intelligence, and no, I don’t see that. So in a way, a lot of effort is being wasted going down that path when we should really be saying, what do we really need for intelligence? But that’s much harder than scaling up an existing idea for VCs to put money at it, for people. If we can publish a paper and we can show the accuracy has gone from 92.3% to 94.1%, then hey, it’s something we can publish and we can get up the HD on that, and we’re making progress. Yeah, that’s how the economy works, but I think people are having this hope, and I don’t have no idea if that’s justified, they have this hope that maybe it’s an emerging property that once we build enough models and we have enough submodels and submodels out of the mind, that one day it will be emerging, this big model, when we talk about this, basically we have an image recognition model that obviously is separate from our neocortex, from the more abstract thinking that once you put the model together. That’s just wishful thinking, I don’t think there’s any evidence, I mean, wishful thinking doesn’t make it so. I mean, there are people who now argue that yes, a million monkeys can ride Shakespeare, and then they cherry pick and say, hey, here’s this big statistical model that wrote something that human people, human judges couldn’t tell the difference between Shakespeare and that. Well, we can see it on Twitter, it’s basically written by AI, well yes, the tweets are not written by AI, but the way we see them distributing through the graph, and that’s, you know, distribution is everything, not just the context, the context is very similar, many, many, many different accounts. Basically all AI and Facebook is written by AI, not the letters itself, but the distribution is done by AI, so it’s working every day already, I don’t know if it’s beneficial to humanity, but it’s a task that was, you know, if you’re actually looking at specific problems that we want to solve in the real world that we need, you know, humans for, I mean, I have a lot of experience in the, you know, customer support area and call centers and so on, you know, talking to people, solving real problems, and, you know, these models don’t get you very far at all, I mean, they, you know, they… I’m with you, when I see chatbots, the first thing I type is agent police, and sometimes it’s immediately recognized, and then you actually get an agent, but very often it’s just you get 15 other questions. Because, you know, the reason is you really require reasoning in the system, you require deep understanding of what the person is saying, and you understand you require real time learning, and what I mean by that, if I, at the beginning of my conversation, if I say, you know, my sister’s moving to Oregon next month, you know, very simple for a human, I mean, it’s like a trivial sentence, you know, but I’ve given you three facts or maybe four facts. That information needs to be integrated, needs to be understood, it needs to be integrated into your world model, and, you know, three sentences later, you expect the person that you’re talking to, to have that information and to use that information, and, you know, the deep learning systems basically don’t operate, they really read only models, you know, you build the model, and essentially it’s a read only model, they can’t learn, they can’t reason, and so to do things in the real world on, you know, problem solving or you want, you want something to autonomously basically solve problems, do tasks or whatever, they can’t. How do you solve this with the current chatbot, and that’s one of the things I think your latest company works on, how do they get an understanding about what someone wants in a specific context, I think the context is very important too, the time of day where the customer comes from, maybe also what product that customer is exposed to, what are the models that work for you currently? Yeah, well, I’m glad you asked me about this because obviously that’s what’s consuming my sort of, that’s my day job and has been for the last, you know, 15 years or so. So the approach that we use is basically the third wave of AI is the cognitive architecture, and the slogan for our current company is basically chatbot with a brain. So there are thousands of chatbots out there, but none of them have a brain. Basically the current approach for chatbots is you use two technologies, you use first wave and second wave. The second wave is for intent identification. So basically if you, you know, use Siri or Lexa, it’s a good example of how that works basically is you can say blah, blah, blah, weather, and you know, it’ll give you the weather report, but you can also say, I hate Uber, don’t ever give me Uber again, and it will trigger the Uber app, you know, because it basically does pattern matching. And it selects one of a hundred or a thousand slots of what your intent is. So that’s the intent identification, which is kind of a one shot thing. It doesn’t take context into account, doesn’t take into account what you said before, what you may know, what you’ve done before. It simply is this is the utterance, that’s therefore it triggers the response. And then the second part of it is first wave technology where somebody and writes a little flowchart type program to basically say, okay, where do you want to go? How many people are going and do you want UberX? And that’s basically how all chatbots work. They don’t have a break. If they work now, yes. So our approach is we have a cognitive engine in the background that basically has a whole ontology of real world entities, it basically has background knowledge, has common sense knowledge of places and people and times and things like that. So when it hears something, it immediately integrates what it’s heard, the new knowledge into that model of the world, into that knowledge graph. And it can then reason about things. Does this make sense? Do I understand it? Is there an ambiguity that I need to clarify? So you have this reasoning engine that is really driving the conversation. So that’s why we call it chatbot with a brain. And now when we talk about the brain, we are nowhere near human level intelligence, but we believe fundamentally the architecture is the right architecture to get us closer and closer to human level intelligence. A system that has deep understanding can learn interactively and can then act on that reasoning. Is it reasonable to have an expectation of a conversation with that chatbot? So when the most chatbots I use, when I have to, I feel like there is, and it’s described as you just did, it’s an if then algorithm, you type in a few keywords and it will come up either with a knowledge base and it comes up with certain helpful links. But I typically feel once I reach that case, I want to interact with someone already screened through the documentation. I did a couple of Google searches and it didn’t get me anywhere. Otherwise, I wouldn’t use the chatbot because I’m conscious of other people’s resources. I don’t want to miss that. So I don’t call my bank to get my balance for instance, I call them an actual problem. I see the balance when I log into the website because that would be my preferred. Right, and I think most people are like that. Is that a real conversation? So let’s do, I want to do an example, but obviously it’s very specific to each use case, but let’s do, I want to, an example in the travel industry, I want to change my flight. I have trouble with that flight, say the airline has already tells me about a specific delay. I want to change it. I just want to know the options that are out there. Is it something that a chatbot could do in a conversation that looks like from my point of view, like there’s a real agent on the other side? Yes, absolutely. And that’s exactly what we’re doing. I mean, on our website, we have an example of comparing a chatbot with a brain in ours is called Igo, company Igo.ai, comparing and we really use Alexa, have, try and have a conversation with Alexa, and then we use our Igo brain connected to Alexa type microphone speaker. And you can see the difference between learning, remembering, reasoning, what, you know, what you would expect. So absolutely what you, what you’re talking about is to have a real conversation. Now the, the difficulty is, and why we are nowhere near human level intelligence. Well, there are several reasons, but one of the key reasons is we as humans just have an incredible amount of common sense knowledge that we just acquire by living in the real world. You know, you know, we know the size of the suitcase or what you can take on board or what you can’t take on board, you know, or whether animals can go on, on a flight or not or what happens when it rains or, you know, just huge amount of information. So to give an AI, all of that background information that you might need for a particular conversation is really, really hard and then to be able to, to use that. So right now to, to have meaningful conversations, we need to make sure that we’ve taught the system, we’ve given it the ontology, the background information that hopefully covers enough of the scenarios of what you’re trying to do. So, you know, if it’s, I mean, we, we’re working with financial institutions, for example, we’re working with healthcare, you know, for diabetes management or so on. But for example, to reset your password in a, in, in, you know, for the bank or for, you know, some critical thing, it actually on average takes people 30 minutes to achieve that. You know, when they, when they, they’re struggling because of the authentication difficulties and so on, then, you know, we’ll send you a key. I didn’t receive it, you know, and, oh, I’m, it’s extremely cumbersome. Yeah. I mean, you know, it’s, it’s like, you’re using the wrong browser, what computer are you using, you know, it’s not working on my system and, and so on. So to give it the right kind of background information that’s required there of, you know, what are computers, what’s a laptop, you know, I’m doing it on my phone. What does an Android and iPhone, I have an old model, you know, just to give it that that, enough of that background information. And then of course you have to integrate it with the company’s back, back end system. You know, so you have to have the APIs, the customer information. So to build these systems is obviously not non trivial, you know, it’s not just a kind of a, a, a plug and play. But the beauty of it is once you have the right architecture, you have a brain, you can then expand and just keep adding to that, that brain. And that’s basically the approach that we have is we have a core knowledge in our brain that we use for all conversations. That’s, you know, how to start a conversation, how to greet somebody, to end a conversation, how to disambiguate. It knows about people, places and so on. So that’s a common core knowledge. And we keep increasing that and make the system, you know, have a bigger and bigger coverage and be more intelligent. But then for given application, you know, whether it’s for, for airline rebooking or airline or hotel or, as I say, diabetes management or banking or helping somebody buy, buy a gift. For that, we, we then have to teach that specific domain knowledge to be able to handle that, that domain. And then in addition to it, the, each individual brain also learns specific customers information. So if you’ve called us, I go before and you’ve spoken to I go before, I go will actually remember what, what you said, you know, that you canceled your flight or you had to delay the flight, you know, or that you’re moving from this town, that town, or you, you know, you always want the middle seat or whatever, you know, it will then remember the previous conversations that had with you, which of course is much better than if you call into a call center because you’re not, you’re not going to be talking to the same person. Or if you, even if you do, they’re not going to remember that the conversation they had before. Yeah, I think it’s, it’s kind of this classic problem, but so when, when the first wave of outsourcing it about 20, 30 years ago, we realized we, we, we spoke to someone on the other side of the world, actually, we didn’t really realize that initially because the company’s trying to hide that fact. Right. Because they didn’t want to make that very visible should be, there’s, to be, we trained Indian cause and the agent with American accents, but we realized even if the accent is perfect because they’re highly smart people, they don’t have the cultural knowledge that we have. Like a lot of words are different, but also they mean different things, even if, if, if the word is the same, right? Even if all the words are perfect, they mean different things, it’s much harder to explain something that anyone would, would know within a minute or two to someone who’s never been to America. I mean, the US doesn’t have that, that’s, yeah, or references like the lake, the lake is just one or what, you know, there’s a storm, there’s a storm in the mid east here in the midwest or something, you know, that right, but what we did is either train them harder or go back onshore, which I think it’s probably a mix with what most companies have. But how, how does the algorithm learn, I’m curious, is it, can you just listen to, to other conversations, or do you have to go into specific models, a bit like an if then model, where you say, well, this is something that we feel is relevant to the specific application. So we kind of make a specific case for it. So there’s a human designer again, that actually makes that decision, or does the system learn from other existing cases that look that they were successful, and then it leads out to ones that were unsuccessful? Yeah. That’s actually a very important question, and unfortunately, it can’t just automatically learn from other conversations. I mean, you know, the problem is, it’s a, it’s kind of a bootstrapping problem. The system isn’t smart enough to know what was a good conversation and what wasn’t a good conversation, or what is relevant in one conversation may not be relevant in another conversation. So if you had the system learn automatically, it would just degenerate very quickly. And we’ve seen that with some chatbots that, you know, they’ve tried self, self learning. So you really need a human in the loop at this point in time to, to, it’s a bit like, you know, the analogy of kids, you know, you have small kids running around, if they just learn from each other without adult supervision, be pretty chaotic pretty quickly. So. I don’t know. You know, that they used to be the kippets that were, were part of the idea was that you basically wouldn’t grow up with your own children. They would grow up with some of the caretakers, right? But they were like 100 kids and two caretakers, and then you would visit them once a month. But they, these kids also grew up fine, right? They didn’t become radicals. I mean, some of them maybe are radicals, but they, they, they, they socialized well and they, they learned what was needed to learn about the real world sooner or later. Oops. Kind of stunning. But even growing up mostly with peers at the same age. Yeah. But mostly, I’m, I’m sure, you know, they, they weren’t just, some grownups were involved for sure. Yes. And, and, and of course our AI is a level that we have, you know, even with, with our, with our architecture and that we, we not at the level of, you know, a young child of general understanding, again, because of this, this lack of general knowledge of growing up in the world. You know, it’s just, the AIs don’t have that. So the interesting fact is that two thirds of our staff in the company are actually not programmers. They are linguists and cognitive psychologists that teach the, teach the system, the knowledge and basically then as we get feedback from actual use cases, we see what additional knowledge do we need to teach it. But at this stage, it needs to be curated. Now, at some point the system will, will become smart enough where it can learn. But then we’re getting very close to what, what you were talking about, learning to earlier, where the system can basically start improving itself first at the software level and then eventually at, you know, the architecture and hardware level. You know, once that model of the real world, right, that’s all these, these, these hidden knowledge, containers of knowledge, once they are mapped out, so to speak, by, by, by just a few, few hundred, maybe a few thousand AIs, then every single AI in the world has that model already. Now they can choose to improve on it or they can just work with what they have. But it’s very different than the humans, right? We have to go through this process all the time and it’s, it’s, it’s a strength to one extent, whether it’s all somewhat unique, but it also takes a lot of time and we could have used for something else. So that’s what I talked about earlier. If we get this model to, to learn on its own autonomously, then it will quickly scale beyond human abilities and just, you could say a matter of years, right? Right. Yes, I mean, that, that is sort of, you know, ultimately my dream and motivation is that we have AIs that are smart enough to help us solve the really hard problems that we, we have, you know, whether it’s, you know, pollution or energy or, you know, poverty or, you know, disease, death, having, you know, as you say, one PhD level researcher trained in, like, say, cancer research, just something that everyone is familiar with, you could not clone that and suddenly have a million PhD level researchers that have all the same knowledge as a starting point and they can go off in, you know, different directions and they’ll also be able to communicate with each other much more easily than human researchers can and they won’t have their egos get in the way either. They’ll have instantaneous access to all the information on the internet, have photographic memory. So the, the, the progress we can make in, in, in, you know, just many areas of, of research is just fantastic. And then I also see us having these AIs as personal assistants and that would be like a little angel on our shoulder, you know, that can give us advice and help us avoid some of the worst mistakes that we make, you know. Yeah. Yeah, I find that fascinating. I always, and it gets, you know, to these, all these topics, once machines get their role, they really give them emotions or will they give themselves emotions because emotions obviously work very well if you can’t solve for all the variables in your equations. Like if you don’t, if you think about diffusion, there’s so many things out there, what, what basic drivers should you have that are irrespective from these really difficult questions? It goes into another topic and you, I don’t know if you’ve thought about that. I sometimes feel that people who are depressed, clinically depressed, they are actually not sick ones. They are the ones who are realists. Right, so we all get into this world, it’s basically suffering, there’s short moments of joy, but they’re given by most of our Olympic system, there’s some serotonin. We all are going to go into this, you know, to the fight and we just don’t know how it’s going to happen. And the people who are joyful and optimistic and want to change the world, people like us, they’re actually the crazy people, we’re just descendants from a lot of crazy people because we are not rational, we are not realistic. We are exuberantly too positive. And I wonder if a machine intelligence will be on the same path or will it, it cannot just be really realistic, right? If there’s a finite lifespan for an individual, if you know about that, we can’t talk to animals because they don’t know that. But if they would know that there’s a death coming, it’s not the best news, right? It’s when you rationally think about it. Why would you use that life to do something great? I think it’s irrational, I feel, but it’s better for us, right? It’s definitely better survival strategy. Well, a couple of things. First of all, I don’t think it’s irrational, in fact, I would argue the opposite. I think it’s totally rational, even knowing that you have a limited lifespan to make the best of it and enjoy it to optimize life, you know, in fact, my personal website is optimal.org. So I think having an optimism and enjoying life, but you know, having an optimism, sort of a proactive, a dynamic optimism that you don’t just optimistic hoping or believing that things will get better, but you actually actively, you know, pursue positive things in life. So you do what you can to realize that optimistic view that you have. And to me, that is the sane and rational way to live life. So I couldn’t come to the conclusion that, you know, being depressed, and I mean, then the logical thing is that you should probably put a bullet through your head, you know, if you want to. But think about all the philosophers. Basically, every grand philosopher was a hermit, deeply depressed, died early. There’s exceptions, right? It’s not everyone, but everyone who was on that level needs to shop an hour. The endless list, they’re all, we would say crazy, but they were all clinically depressed, not all, 99% of them. It can be coincidence, I feel, or maybe just if they were chosen, right, because maybe they were depressed, that’s the only thing they could, they could, they could excel at. You could make that argument, maybe, but I keep thinking that I’m a very optimistic person that I’m just trying to say sometimes. Maybe I also don’t resonate very well with, or I don’t think I got very much out of those philosophers. Okay. Now, maybe they sell well. I mean, Bertrand Russell is one of my favorite philosophers and, you know, he lived a ripe old age, and I think he certainly didn’t go through life being depressed as far as I could tell. So Ayn Rand was also a philosopher that I think I got a lot of inspiration from and, you know, very, very positive view of what we should achieve as, can achieve and should achieve as humans. But I do want to just remark quickly on, you know, you say that we know as a fact that we’re going to die. Well, there are people working on extending life very significantly, making indebted lifepans. So even that isn’t, you know, like death and taxes are the two sure things in life. Well, I think most of them could probably be dealt with with human ingenuity. It’s time for it. Yes, we had Robert DeGray on, you know, me and David Sinclair, they’re kind of spearheading this effort right now. And he was extremely confident that this is something that’s going to happen in the next 15 to 20 years. Singularity. I want to ask you about the singularity. Do you think it’s going to happen? Do you think it’s going to happen in 2038? Ray is very specific by now. And how do you think it’s going to look like? Obviously, we call it singularity because it’s hard to predict, but what do you feel if it happens? How will it look like? Yeah. So as I mentioned earlier, you know, you said that when computers can design themselves or improve themselves, then clearly we’ll see an explosion of that artificial intelligence. I don’t see any reason why that wouldn’t happen. I mean, if we can build machines that can, you know, give comprehensive customer support, you know, and really at human level of deep understanding, there’s no reason why we couldn’t have computers that can be researchers. And if they can be researchers, they can be engineers. And in fact, I kind of half jokingly say, you know, and people ask me how, you know, how long do I want to continue running AI companies and so on. I say, well, when my AI is smarter than me, when it’ll take my job, then I’ll basically stop doing this. And it can happen one of two ways, either I can get dumber and dumber, or the AI can get smarter and smarter. So whenever we have that crossover. So yes, I believe computers will become software engineers, they will become hardware engineers, and basically they will be able to understand their own architecture and will be able to improve on that architecture. And they’ll be able to do that at a much faster cadence than humans can iterate much quicker. So that to me is a singularity when computers reach a truly human level intelligence at a general level, not at a narrow level, and we’ll see that explosion. Now how that will change humanity, I mean, one of the things that if you ask most people, would they like to win the lottery, they’re not many people who will say no, I wouldn’t. In fact, I don’t know, anybody would say that unless they’ve already won the lottery and it ruined their lives, you know. But you know, that’s really where what the singularity will bring, we will no longer have to work. So we all win the lottery, right? We all win the lottery, we basically will have, you know, it’s actually an interesting analogy, you know, we talk about it. The race should sell it this way, they’ll be all going to win the lottery and live forever. It would be very convincing. Yeah, yeah, indefinite lifespans, we live for as long as we want to live. You know, it’s kind of an interesting analogy, we talk about some of you, they live like a king, you know, now, but we go back and see how kings really lived, you know, hundreds of years ago. Yeah, it’s not very good. We live like kings, much better than kings now, you know. And so you go forward to having this radical abundance where, you know, really the material needs that we have become trivial to provide for everyone. Then of course the focus changes on how do we have a meaningful existence, what do we want to do with our lives. But again, their AI can help us, because we’ll have AI’s that are better psychologists than human psychologists that can help us, you know, decide what we want to do with our lives as humans. So I see that as a very positive future with AI, but it will be very different and people who obviously resist change will struggle with that. And then the question is, can those people who don’t want to change, can they continue in sort of more or less the same life that they’re having now? And perhaps, perhaps that’s the answer, I don’t know, you know, like the Amish live, you know. Well, they live very, I think they live very well, right? So they certainly have a different living standard, but they certainly live well in the sense of their own happiness, at least seen from the outside. We only don’t know what’s going on on the inside. So whether we’ll have people that want to just kind of be frozen in sort of 21st century life, but, you know, not really having to worry about, you know, material comforts so much. I don’t know, of course, there’s a problem of addiction, but again, we’ll have AI psychologists that help us, you know, gaming addiction and things like that. It’s kind of hard to say where our role is, right? So because it seems like whatever we are good at sooner or later, we will teach AI to be good at this too. And then maybe there will be this one designer left, right? So like you just said, you design so many of those AI babies, so to speak, but sooner or later, these students will outgrow the master. And then, well, the master is not needed anymore, right? So this is generation of humans will become, might be pretty lonely, right? Because humans are not really needed in their physical appearance and in their limited physical experience, they’re not really needed anymore. That’s I think a bit of a fear on one hand, we all in the lottery and we live the best life ever. We are dimensionally rich compared to today, but we really don’t, we are not really needed anymore. And to the other, I mean, we don’t really know where we are on this planet, right? So it’s kind of a, what is a meaningful life changes all the time anyways, 500 years ago, the answer would have been completely different than what it is now. Yeah. And it’s, I mean, I look forward to that there’s so many things I’d like to do and like to explore apart from what I’m doing now. But yes, how well we will manage with this additional freedom that we have, this radical freedom that we’ll have will be, will be interesting. But to back… Do you think it’s in our lifetime? So it’ll be in the next 20 years, this massive change, or do you feel like this is going to be more like 200 years, 2,000 years? Yeah. I always answer that question in terms of, I don’t think it’s, it’s as much a question of time as a question of money, is the right money going to go into the right approaches of AI? We could have trillions of dollars, in my opinion, we could have trillions of dollars going into deep learning, machine learning, and 50 years from now we still wouldn’t have general AI. You know, can we tear ourselves away? Can we go back and really say what do we need for intelligence and start focusing on that and building systems that have intelligence? Given the right kind of effort, I believe we can have human level AI in definitely less than 20 years. That’s very optimistic. That’s great. It’s very interesting that you say that very few people who come on the podcast who are generally very optimistic about narrow AI, kind of because it worked so well the last 10 years, but very pessimistic in any approach about human level AI, it’s more than 5,000, 600,000 years away. That’s kind of seems to sentiment for most people on the podcast. Yeah, of course, if you don’t understand what the problem is with the current AI’s, you can see the limitations. You can see there is no real intelligence. I think people sense that, you know, even with the magical things that deep learning machine learning can do, people still feel, no, there’s nobody at home. This isn’t real intelligence. If you don’t understand why that is, you could easily say, well, we have no idea. It could be hundreds or thousands of years away before we crack it. If you actually understand what the problem is with the current approach, that we need a third wave approach, then you can say, okay, what do we know? What don’t we know? What can we do? What can’t we do? You know, and I now have 20 years of hands on experience in building these systems with a relatively small team, well, very small team, you know, I mean, Google, Amazon, I believe has 10,000 people working on Alexa, I was told. Oh my gosh. I mean, the mind boggles, you know, and, you know, we have a team, we now have a team of 25 people, and typically we’ve had like 10 people. But this is where innovation comes from. Mind is just smaller teams. Once you’re that big, it’s almost impossible to get really innovation to work. Sometimes you get lucky, but it’s really, really rare. So what do you think is the limiting factor? Because money usually follows innovation, right? So obviously it takes a while to scale up and get critical mass, but the VC dollars sooner or later arrive at the most innovative spare heads in this whole economy. Do you think it’s really just money? So like in five years from now, it’s going to be trillions in a third wave AI approach, or what’s really stopping? Or is it the engineers that have to rethink? Yeah. So, I mean, VC money follows trends typically, you know, I mean, they jump on, they jump on bandwagons, you know, and yes, it’s, and it’s short term thinking. So, so it’s, I don’t know where the best funding is obviously we ourselves have had a lot of experience in talking to different people that, you know, want to fund it, but typically VCs and investors are always just, they may not ask the question, but the question is always what’s your exit? You know, basically, when am I going to cash out? And with that kind of mindset, you can’t build fundamentally new technology. You know, it’s, it’s, you really need more of a vision, especially if it’s something as hard as like what Aubrey de Grey is trying to do, or what we are trying to do is to build human level intelligence. So I think it will come to a point where the third wave of AI will have enough examples, real world examples of where it’s actually working, solving problems that you can’t solve with other technology. And we, we believe we’re on the cusp of that. And we do think then that, you know, there will be kind of a, an explosion of interest in this field. But it, you know, at the moment, VCs ask their AI experts to, you know, look at a system and their AI experts are all deep learning machine learning experts. That’s the only thing they know, and that’s the only thing they can judge things by. So yeah, the limiting factor right now is the number of people working in on the third wave. It’s minimal. There’s hardly anybody working on it. And you just need some more people. You don’t need, you know, I don’t think you need hundreds of thousands of people. You don’t need trillions of dollars, but you need more than a dozen people working on it. Yeah. Aubrey de Grey told me how difficult it was for him to initially raise funding because it seems so outlined, I should say. So we’ve heard this all before, and why should we give you money in it? He says, you know, it’s really changed the last two or three years of money is pouring in almost too much. They don’t, some, some applications are so advanced, they don’t really need that much money. But he felt like his fundraising target, you know, obviously keeps changing. And once this industry actually starts to work, it will all change again. But you can do a lot of like a few billion dollars. You can, you can change how long we live, which is a huge target. But he said with 10, 20, 30 million billion dollars, that’s enough to really drive it to almost, you know, practical, not just practical, but in a sense of you won’t have a drug that will, will be able to go to marketing, maybe for this, but generally this is enough money to really research that subject and hopefully bring it to fruition. And that seems to be doable, right? And raising a billion dollars, we’re giving how much we spend on infrastructure or anything these days, a billion dollars seems like a decent fundraising card. Right. But, but people who can write billion dollar checks, you know, would need to understand that would need to come to the conclusion that the third wave is, is to invest. It’s imminent. Yes. And, and then of course, even if they believe in a third wave, I mean, billions of dollars have actually been thrown at this before by DARPA and different government organizations. But, you know, they’re incredibly inefficient, of course, because they end up giving, you know, 30, 30 million to this university, 20 million to that university, and, you know, the money just disappears in administration and whatever projects they have. But yes, it’s, it, I would agree a few billion dollars absolutely would, in my mind, unquestionably would, would put us to a point where we can see yes, this third wave cognitive architecture approach is really, you know, going to get us somewhere. But it’s, you know, it’s getting, getting that point. I mean, Google DeepMind has already spent several billion of dollars, you know, they have, I think they’re burning through 600 million a year or something, haven’t, I haven’t actually seen much output from them at all in the last few years. So I don’t know, I don’t know what it was. It’s kind of like what we thought of AI in the 60s, 70s, 80s, 90s, right? So it makes these big jumps, something works, and then it’s really quiet for a long time and I’m like, man, do we have to give up on this? And then, well, that’s deep learning, right, it came out of nowhere. Right, right. But deep learning is sort of sucking all the oxygen out of the air, you know, in terms of any other approaches. And I can give you one anecdote or example here. We had a brilliant intern from Germany work on our project. And then he went back to Germany to do his PhD. And now he was totally sold on the idea of third wave cognitive architecture approach. You couldn’t find a sponsor for it. So he ended up doing his PhD in deep learning machine learning. So here’s another researcher that could have moved the technology forward. He’s lost now because he’s not going to get it, you know, what’s, where’s he going to work or what’s he going to, if he stays in an academia, he’s going to teach deep learning machine learning. If he goes working for a company, he’ll be working on deep learning machine learning, you know. So it’s, it really is not going to be ahead of your time. I noticed that too. It’s very tempting sometimes, and it’s great to be right. But it’s, it’s not, well, you, you, you’re going to be more, you’ve got to define yourself more like an artist. You, as an entrepreneur, it’s really hard because you just need that buy it, right? That needs to be a marketplace that wants your product or as someone who was looking for a job. But if you’re fundraising, if you’re too far away from a future market, it’s just not much you can do. You can only wait and hope that it takes up in five years and then you’re in that, and that’s really. So fortunately, now in our commercial company, we are now at a point where we have a product, you know, a chatbot with a brain, we have the architecture, we have the infrastructure, we have customers, we have references. In fact, my previous company has now been in operation with the first generation of this technology has now been in operation and, and, you know, profitable, has been in operation since 2008. So we have, we have a lot of experience at companies called Smart Action, and that’s an automating phone calls, basically, in the call center. My new company, Igo.ai, is focusing on chatbots, on text interactions. And so we are fortunate in the, in, where we now at a point where we are solving real problems in the real world and, you know, generating value. And so we see that we, we don’t just have to kind of, you know, be in our shell and do our research and hope that the time will come, you know, or consider ourselves artists. Well, I think humanity deserves to, to get where we, you know, we pointed at earlier that we’ll, we’ll be there in less than 20 years at a, at a level where we feel we can recruit AIs, right? So instead of 9 billion people with very different profile, but we, we get to 100 billion individual intelligences that can help us solve the problems that we have and it can scale further on. So we need free energy to get to, to, to other stars, right? So we’re almost free energy and massive amounts of energy and we haven’t solved it in the last, we haven’t made a lot of progress in the last 50, 60 years. So I hope you’re right. I hope we’re going to see this in less than 20 years and I hope this third wave AI will make a lot of progress in the next couple of years. Peter, thanks for being on the podcast. That was awesome. Thanks for the update. Great. Okay. Well, thanks for having me. I learned a lot. I hope we’re going to, we get to the talk again. Great. Thank you. Bye. Thank you. Take it easy. Bye.