Matei Zatreanu (How alternative data is changing the world of finance?)
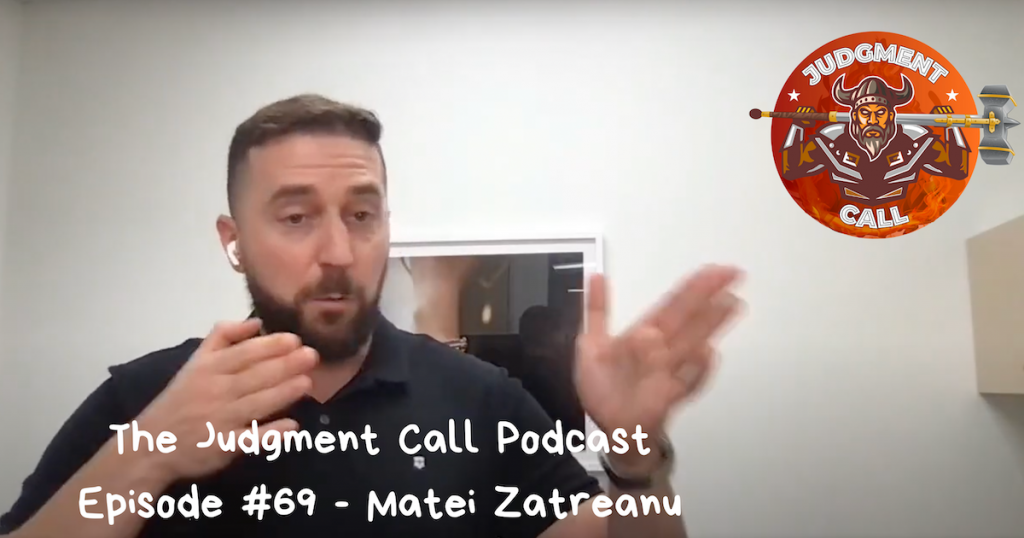
- 00:01:43 How Systems2 discovered a world of alternate data and how financial data companies in this space operate?
- 00:12:23 Can there be a profitable Open Source investment community?
- 00:25:53 How much time is spent developing algorithms or cleaning datasets in Matei’s work?
- 00:34:11 What are surprising and low cost datasets one can acquire?
- 00:44:20 How good are predictions for actions of individuals today? Does Facebook and Google know everything about us?
- 00:58:20 Why antagonism is a great way to learn about your strength?
- And much more!
You may watch this episode on Youtube – #68 Matei Zatreanu (How alternative data is changing the world of finance?).
Matei Zatreanu is a quant, investor and entrepreneur and now heads System2.
Welcome to the Judgment Call Podcast, a podcast where I bring together some of the most curious minds on the planet. Risk takers, adventurers, travelers, investors, entrepreneurs and simply mindbogglers. To find all episodes of this show, simply go to Spotify, iTunes or YouTube or go to our website judgmentcallpodcast.com. If you like this show, please consider leaving a review on iTunes or subscribe to us on YouTube. This episode of the Judgment Call Podcast is sponsored by Mighty Travels Premium. Full disclosure, this is my business. We do at Mighty Travels Premium is to find the airfare deals that you really want. Thousands of subscribers have saved up to 95% in the airfare. Those include $150 round trip tickets to Hawaii for many cities in the US or $600 life let tickets in business class from the US to Asia or $100 business class life let tickets from Africa round trip all the way to Asia. In case you didn’t know, about half the world is open for business again and accepts travelers. Most of those countries are in South America, Africa and Eastern Europe. To try out Mighty Travels Premium, go to mightytravels.com slash MTP or if that’s too many levels for you, simply go to MTP, the number four and the letter you.com to sign up for your 30 day free trial. I like how you say that and now it’s settled. Yeah, I love it. I love it. I love it. Thank you for coming on the podcast. I really appreciate that. Thanks for being here. Thanks for having me. Hey, absolutely. And I really appreciate your background. You’ve been a quant. You’ve worked in the financial industry and now you’re an entrepreneur. You’re running your own startup system too. And from what I understand, it’s all about alternative data and how this massive amount of data can be used to make companies more efficient and make companies better and make more money also. Maybe you can tell us a little bit about your company and your background. Yeah, I’d love to. So I start off in relatively traditional finance environment. I start off at an investment bank and after that, this was Lehman Brothers in 2008. They went under during the last crisis. It’s kind of funny because now you have to specify which crisis it was. It used to be enough to just say like, oh, during the financial crisis and now that we’ve lived through multiple once in a lifetime crises, you have to kind of specify. But after that, I end up at a hedge fund where it was, I ended up spending almost a decade at this fund. And my role was, from an educational perspective, my background is in math and stats. But this fund was very traditional and had humans that were making these decisions about the companies to invest in. A lot of those decisions were made sort of qualitative. I mean, obviously, there’s a lot of information, a lot of data that was consumed, but it was done in the more ad hoc manner by individual human beings. And I ultimately ended up starting a data team, data initiative for this fund that was responsible for answering. I figured out what kind of questions would we even want to understand, an ideal world? If you can have an answer to any question, like I was like, Google 2.0, and you can know anything about the companies that you’re looking to invest in, what would you want to know? That started off this waterfall, this cascade of searching for different kinds of data sets that they can answer some of these questions that, beforehand, could not be answered. So, for example, and we can get into this later, but we started having access to things like credit card data. So, seeing consumers transactions on a daily basis, like some percentage of the consumers transactions, and that gives us insights into how companies are performing. We have data for everything from web traffic to location to all sorts of very niche data sets. For example, the mining industry or the travel industry that are really good to answer those kinds of problems. And about five years ago, I decided to leave the fund because I became convinced that the future of investing was going to be data driven, and I wanted to start a company that can really make that happen. So, we started System 2, and the idea behind System 2 is that we become the data team for all sorts of companies. It started off being mostly investors for no better reason than that’s just the world that we came from, who don’t have an in house data team, but want to leverage some of these data sets and the expertise that is required to make sense of it in order to solve real business problems. We’re not academics, we’re not here to write some interesting hypothetical paper on some obscure insight that we’ve found. We have to find something that’s actionable. So, being actionable is really critical. And in May, over the time of expanding, we now work with corporates. I mean, the demand for not necessarily data, because people don’t really care about data, it’s the demand for insights. The answers to these questions that up until now were impossible to answer is just exploding, and having that kind of a skill set and ability to deliver these kinds of insights is becoming more and more popular. Yeah, you sound like the person who’s up for the job. It’s pretty amazing. And when I did some research about the company, indeed, I didn’t find a whole host of white papers. There were a bunch of podcasts you were on. And that seems to be it. So, we noticed from a lot of Quan shops, hedge funds, they’re extremely secretive. They’re kind of like Apple. So, everyone who even goes out and mentions a little detail gets sued or changes employers. And that seems to be working for them, right? So, they seem to be in this particular niche of innovation that they have mastered. So, it’s probably a very small secret. That’s why they have to guard it so much. But they’ve been very profitable. Renaissance has been extremely profitable on a data driven investment mindset. How do you see the investment industry right now? There’s been data driven, at least we recognize them. I don’t know how Renaissance actually works. There’s been data driven hedge funds for quite some times. They haven’t done so well in the last two or three years, unfortunately, or maybe fortunately, that depends on your perspective. How do you see this world evolving? Yeah, that’s a great question. So, just to kind of unpack the questions a little bit. So, in terms of the secrecy angle, you’re absolutely right. I mean, if you look at even our own website, it has very little information on it. And that’s again, just a byproduct of the hedge fund legacy. If you go on the websites of pretty much every single hedge fund, all you’re going to see is just a splash page saying like, yes, we are this hedge fund. And there’s just a ligand. So, if you’re an investor in the fund, you get to ligand. But otherwise, there’s absolutely nothing on these pages, except for maybe disclaimer. Because you have to have all of them. It’s good for them to maintain any infrastructure to deal with their stakeholders and shareholders. Well, I mean, that is true. There’s definitely no need to deal with it on a public level. But there is definitely a ton of stuff behind the scenes that you have to do reporting. And there’s a lot of, there is actually a decent amount of infrastructure that’s built the report to the end clients or the investors of these hedge funds. But that’s partly driven by regulation. So, there are all sorts of rules around how hedge funds can market or how investment firms in general can market the people. So, to be safe and to be conservative, they haven’t done that. Having said that, there is this, it is actually more collaborative than it might seem. There are a lot of conversations that are happening, but they’re happening behind more closed doors or said another way they’re happening through old school mechanisms, like picking up the phone and calling. So, before analysts at a hedge fund, that again, it’s very secretive. And I’m talking right now, I’m not talking about the quant investors, because there’s, there are two classes, there are the fundamental investors that still have people in the mix, you know, think of like the warm buffets of the world that are really deep, deep down in the fundamentals and thinking about these companies, then you have like quants on the other side that have machines and computers doing all the trading. So, I’m going to focus on the fundamental investors to start. They still, before they make these kinds of investments, they pick up the phone, they call up the cell sites. The cell sites are these, you know, the banks that put out research papers, they’ll see what is known out there, because it’s not enough for you to have a view about a company, but you have to have a differentiated view. If everyone else knows the same thing that you know, you have nothing, you have no, everything’s already priced into the stock prices. And so, there is a lot of collaboration that is happening. But at the same time, yeah, it is just old school, like picking up the phone, calling your friends that you have other, you know, friends that are hedge funds or friends on the cell side. And there are entire like companies and ecosystems that have sprung up around this, right? There’s the whole expert networks that their whole business model was to put you in touch with an expert who knows something about a certain company. But yeah, I think overall you are right. It is much more secretive and people do feel like they have something proprietary. To be honest, that’s never been my view. I mean, I think the reality is like everybody’s especially in the finance world, people think that they’re like the smartest people in the room, like everybody thinks that. And when you, and it’s also like everyone’s above average, right? Everyone’s above, of course, absolutely. And the reality is like there are a lot of smart people that are obviously working at other hedge funds and even in other industries. And so there is this benefit from being a little bit more collaborative. And what’s been happening recently with the rise in like fente companies and companies that are working on these alternative data sets, that there definitely has been a lot more communication and there are more white papers. There are more, like it used to be enough, like when I first started doing this, it was around like 2014, just knowing that a certain data set existed was like huge. Very few people knew that you can buy credit card data. And then if you happen to know who the vendor was and you had access to that data set, you can make some really interesting trades. Now, like all the incentives point in the direction that the vendors want to sell to as many people as possible. And what ends up happening too is that people will just leave their jobs and it’s like this constant like rotating wheel of who’s where. And that just leads to more information getting disseminated. So the reality is like, and this is where we come on on these issues is that it’s not so much about the information advantage. I mean, there is usually an information advantage, but it’s usually for a very limited period of time. After that, it’s much more about the analyses and just rolling up your sleeves and doing the hard work of analyzing these companies. And so that’s sort of the secrecy angle. And you were talking about some of the quants like Renaissance and others, like their approach, like, yeah, they’ve been using data for a very long time. But to define our terms a little bit more sharply, the data that’s been used in finance for many decades is like the traditional data, compared to alternative data, the traditional data tends to be more around prices. So how much are what is the stock price, right? This is a very simple question. But if you actually break that down into what it how you arrive at a stock price, it actually is a very complicated question. Because there’s a market out there, right, where people are constantly trading these securities and there are prices for every single, every single trade. But these trades can happen in very small increments. And so like just to give you a simple example, like, well, okay, do you take the average price over a certain hour, like if you break the day up into hours, and you say, okay, what’s the average price of all the trades happening in that hour? Like that sounds like a very reasonable way to find out the average price of something. But you might have a situation where during lunchtime, like nobody’s trading, or if there are very few trades, and there are certain hours where there’s a lot of volume. So now let’s say, okay, well, maybe I want to place a higher emphasis on the prices where a lot of stuff has transacted versus like some one off guy who has happened to really want this stock during lunchtime, and he paid a lot of money for it. That doesn’t necessarily mean it’s not reflective of what’s what the real prices of these securities. And so these traditional like quant folks spent a ton of time analyzing very granular like price and volume data, and trying to find patterns within that price and volume data. And there’s obviously there’s some other information that gets layered in there, but that was the bread and butter of it is being really good and trying to find these patterns on various granular scales, but across like tons of, you know, like long periods of time across tons of different securities. Yeah, it seems to be, I looked into that some time ago, and it seems to be a spark of I’d say an open source hedge fund. I’d say movement out there and there is alpaca, which is which is a broker where you can run your algos. There’s been wealth fund, I think they started with something similar, but they moved away from that business model. And there’s a lot of stuff on like code repositories like GitHub where you can basically download see what works for you. You can play with it, different AI strategies, and you can put in any data you want. But I fully agree. Typically, it’s still the same kind of data, right? It’s pricing data, it’s time series from different pricing movements. It’s volume data. And it’s it’s, it’s kind of boring data. It’s from what I’ve been told, there is really no music in that anymore, because so many people run their algos on it. And it’s just unless you have some incredible insight, there’s a very hard to make money with this, especially in markets. And you said that earlier, where we have, I think a financial term, where it’s a one in a lifetime, I think there’s a better term with a standard deviation. What is it? You probably know the the once in a lifetime crisis, which should only happen once in a lifetime happens every five years now, strangely, right? Because well, because we built these incentives, like we moved so much money on the other side of the scale, that’s why we have the sudden crisis is the the open source community seems to be up to something. But obviously, you said that earlier is if once a certain amount of people have the same data, then your edge goes away and you can’t make money. So the open source investing in that sense, if it is exploiting a certain data stream is probably it’s an oxygen mode is probably never going to work. But I think yeah, I think it’s it’s a fair point. And it’s, it’s just a matter of timing, like it can work. But for some amount of time before other people pile on to it, I think that’s one of the cool things about finance and what initially drew me to this is that what we’re if we abstract away from our day jobs, what it is that we’re ultimately doing is making predictions. And people make predictions in all areas of life. And finance is one of those few areas where you can actually figure out if you’re right or wrong. And there’s been a ton of work by my folks like like Tetlock, who have studied this phenomenon of like what makes a good forecaster. The problem is that you see this all the time on TV, where different pundits are making some kind of like things that are essentially forecast, but they’re done in such a hazy way, that’s really hard to pin them down to either they don’t specify timing. So it’s like, yes, I predicted whatever this is going to this is going to happen. But and it has 50% chance that the market goes up tomorrow. And it goes down the stunts. It’s a, it takes a moment for the non expert to parse and realize it’s all complete bogus, right? Do you absolutely right? Exactly. And there are other places like, you know, games like poker where people are making some kind of decisions and you’re, you’re trying to read your, your opponents and try to make essentially forecasts about what do you think is going to happen to the cards that are being dealt. And, but finance is a cool opportunity because you can make forecasts about so many things in the world. So many different companies, so many countries, so many different, like probably what have you, there’s a way to express your belief and a way for you to find out whether you’re right or wrong. So I think that component of it is really interesting. And so going back to like the open source movement, or if there are people that are trying to democratize this, it’s, it’s definitely possible, but it is one of those things where when somebody else, when a bunch of other people figure out the same thing as you, the, the, the entire alpha, like the edge that you might have had before goes away. And we can talk about this more as well, but what’s been happening recently that’s kind of interesting is the whole Wall Street best phenomenon, like the Reddit forums where people are getting interested in investing and they’re collaborating, but collaborating in a very different framework. It’s no longer like collaborating with the, your coworkers, because they, they’re independent traders now collaborating within these forums. And a bunch of this stuff has moved to other places. I mean, there are Discord, if you’re familiar with Discord, it’s like a Slack app that’s for, for gamers, typically, but now they have all sorts of these like closed essential servers that you have to apply the, the join into and they have to let you in or not. And once you’re inside, people are discussing these kinds of ideas and they’re sharing this kind of, this kind of research. And so there are a lot of, a lot of ways to collaborate and to share these kinds of insights, but some of the, the traditional ways of making money are still true, right? You have to know something that somebody else doesn’t know. And the other side of it too is that you, you may be right about something, but like I mentioned earlier, the timing perspective is huge. And so like, you know, the old joke is that you can, someone’s ability to, to be right is, is, doesn’t matter because you’re ultimately outweighed by someone’s ability to, to stay solvent, to stay in the market. So it’s, there’s a lot that goes on into it. And it’s, it’s kind of cool because you’re, you are making these forecasts, but at the same time, you’re also playing a game essentially against other people and trying to figure out what other people might not, might not know. Yeah, the timing aspect is usually what gets me. I feel like my forecasts are usually correct, but I’m like five, 10, my time period is completely off. So my market timing is horrific. And I, I usually get out of an investment and then it goes that way sooner or later, but it’s like five years later. So I’m, I’m, I’m terrible at this. And well, I was, I was chatting with Jim Rogers a few weeks ago. And he’s been, he’s been saying that’s, that’s still with all the knowledge he has accumulated. He’s one of the best investors we know. And he definitely is one of the most public ones. And he said, well, market timing is impossible. You can’t sometimes predict this catalyst, right? This is this moment when things actually change and they fall into place when you predict it all the time, but it might take 20 more years until this catalyst actually comes along. And you have to be extremely patient of it for these things. And by the time maybe your whole investment hypothesis, you might have forgotten about it because it’s been 20 years. I don’t really remember what I thought 20 years ago, about the next 20 years, right? So it’s quite challenging. But you’ve been, and that’s what you do with your company, you go into a slightly different approach, right? So alternative data basically means you use data that basically has nothing to do with the financial industry, like credit card data, or through this, I would count that still as the financial industry, but it could be hotel occupancy, or it could be, I don’t know, traffic data, for instance, location data. And you take that data, you massage it, run it through algorithms, and then have the machines and the analyst make predictions in order to, and you use this knowledge to trade yourself or you sell it to hedge funds. How does this work? Yeah, so right now we are not, we’re not trading ourselves, like we are answering questions that our clients come to us with. And so hedge funds or financial clients will have these kinds of questions about a certain company that they’re looking to invest in. And the really, I think that the main differentiator between what the fundamental analysts do versus the quads, the quads, again, are just trying to find these like high level patterns in data, usually pricing data. Fundamental analysts are trying to find a story. So why is a certain company doing what it’s doing? Why is Peloton succeeding? And, you know, you can have all sorts of stories, like obviously with COVID, people are staying home, they’re no longer going to gyms, they’re going to buy a peloton for their house. But then you would come up with so many, like once you start forming this, this story, there’s so many different possible paths with that story. And now you’re saying, what happens when gyms reopen and people get vaccinated? Are they going to go back to the gym or are they going to like their peloton so much that they are changing their behavior and sticking with that? And so once you have these kinds of questions, you can then try to figure out, okay, what kind of data set could I use to help me answer these kinds of questions? Like you mentioned, these data sets are, can be anything. And the only way to think about it is that there are so many companies out there doing their just regular day to day business operations. But in that process, they’re collecting information. And that information can be and usually is valuable to people like us who are trying to understand something about that industry. So whether it’s, you know, a company that works with internet service providers, and they’re providing some kind of, I don’t know, some sort of service to those internet service providers in exchange, they’re collecting data about traffic to websites. Or it’s you mentioned, you know, like hotels, there are companies that are working with the hotel, the airlines, all these different, different components that can decide to essentially like sell their data in order to give folks like us access to it so we can answer these kinds of questions. So it’s an ability to, first of all, to think much more deeply about the questions that we’re trying to answer. And then almost, it’s almost like we’re detectives, right? Okay, this is where we’re trying to figure out like, what is out there? What might be out there that can help us answer this question? And how do we go about getting that data? And there’s an entire process that involves like, first of all, like, let’s figure out what is we’re trying to solve? Like, what are some hypotheses of ways we can, we can try to solve this? And then how do we find the data? It used to be the case that you had to pick up the phone and call. So for example, there are companies that provide expense services. So if you send in pictures of your receipts, and they help you with your accounting and expense management, and we realize, okay, some of those, those companies have data on on travel plans. So if you’re an employee of a company, you have to submit your expenses, and it’s usually run by some of these companies. So why not give them a call and see if they would be willing to provide us with, you know, anonymized access to this data, so that we can figure out like, which rental car companies are people choosing, which flights, which hotels, and, and that way we can answer these kinds of questions. But over time, what’s happened is that more of these, these companies, like there’s been an entire ecosystem, or marketplace where there are now brokers that can help you find data, they’re more companies that are trying to actively sell their data. So it’s no longer such a such a search that you have to undergo, it’s much more of, okay, let’s figure out which of these items, data sets from a menu we want to choose. And that’s only that’s only the beginning. So once you have the data, there’s an entire like engineering analysis process, because all these data sets are massively flawed. And our job is to figure out like, how, how much do they suck? I mean, that’s the one sort of like one terrible part of our job is that you’re talking to anybody who’s trying to sell data, and their incentivize to tell you like, this is the most fantastic data that you’ll ever see. The reality is it’s all terrible. It’s just the question of how terrible is it? And can we identify where, where is it terrible? Because once we know that, then we can start to figure out, okay, how can we fix some of the issues that that the data has. And the main one being is that we don’t, we never see all of the data. It’s not like this is, you know, those are even like the pricing data that we discussed, where you have a market that actively trades stocks, you can see all the information that’s happening on that market, even that, by the way, took some time until they got the kind of disclosures and transparencies that investors really wanted. But here it’s like, okay, I’m on this, you know, this company that handles receipts, and I only see maybe 1% of all the bookings for this airline or this hotel chain. And you can imagine that 1%, by the way, 1% is an average percentage that we see in terms of some of these, some of these data sets. And so we have to make do with just like 1% of all the customers. And how do we extrapolate from that, try to figure out what’s happening to the rest of them. And by the way, that 1% is that’s another really terrible thing is that it’s never static, like these, these companies, these data companies are constantly adding new sources to their panels, they’re losing access to some of their sources. So it keeps churning. And that churn causes a lot of a lot of issues. So there’s a, we spend the vast majority of our time just trying to deal with all this like statistical nightmare of what’s happening in the real world of data. And it’s super messy. And there’s, there’s tons like business negotiation terms, like things that fall south, like gossip, all these, you know, we heard that these guys are about to lose one of their main suppliers, like what are we doing that case, how do we reposition entire engineering efforts in the chance that yes, we’re about to lose one of these like major components of our data sets. And then ultimately not losing track of the fact that we need to come up with good forecasts for, for the questions we’re trying to answer. This sounds fascinating. And one example that, that I personally, or personally from the media, I’m aware of is there were a bunch of Chinese companies who were listed at the NASDAQ and they claimed they have a certain factory output. And they had a certain amount of customers and there are a certain amount of business basically. And then based on satellite imagery, there were basically nobody ever went in or out the factory. And that was a clear sign that this business is not doing so well and might hide some facts. And I, I found that a genius idea. Maybe, maybe you guys would have thought of that immediately. I thought, well, this is a quite a difficult connection to make that you apply some satellite custom satellite imagery, because you need to change the amount over a couple of hours. When we look at this, I’ve heard this from, from other people who deal with big data that used to be the, the, the name before now it’s moved more into AI, right? A lot of people have claimed that this is often the biggest problem is to, to clean up the data sets. And then there’s a bunch of algorithms that basically you just download from GitHub or wherever you download them from, right? This is almost like TensorFlow gives you a huge library of potential algorithms that could show you patterns in the data that you see. When you, when you look at the challenges, and I think you just outlined that, is the algorithms that you employ in order to find patterns, is that a big deal or is 90% of the work is really massaging the data? Yeah, that’s a, that’s a great question. And it’s, the answer is, is more complex when you, when you just go into the weeds of it. So algorithms, I mean, that’s been the, the sexy thing, right? Everyone’s talking about AI and machine learning. And there have been tons of companies that have raised a lot of VC money because they claim that they do all this amazing machine learning stuff. And you’re absolutely right. A lot of the actual math behind it is publicly available because it tends to come out or has been coming out of academic institutions that are just putting up the, the papers online for people to, to implement. And a lot of code is already written, like you mentioned, that you can download these libraries and Python from, from, you know, your favorite repository and you can implement this into your code. So that has been more commoditized. I mean, when you go to the edges, you will find examples like, yeah, I guess the companies that have an advantage on the algorithmic side can do things like process data faster, maybe come up with an algorithm that’s more efficient than the code that you can run there. And yeah, maybe that makes, makes sense if you’re trying to, if you’re a massive company and you’re trying to analyze tons of images, for example, and you, any improvement in the speed of that processing power, it makes a, makes a huge difference. But for most of the things that we’re talking about, like I mentioned earlier, like, most of the time we’re only seeing a couple of percentage points of the transactions out there in a certain domain. And even those can be significant. But the challenge isn’t really speed. Like we have plenty of time. Like we never butt into processing power or speed issues when we’re running into challenges. It’s much more the, the, the formal point that you’re making is around the quality of the data. And honestly, the only way to solve that is, is not, in my opinion, it’s not through better modeling. So I’ll give you a perfect example. We’re actually talking about this in our company this, this week, where you can have and try to make a forecast on something. And one of the, one of, one of us shows a result saying, okay, like this model works so much better than this other model. And your instinct might be, okay, great, fine, let’s just like show this to the client. But what you really want to do is just say, okay, let’s take a step back and try to understand why is that one model working better than the other one. And this goes back to just my personal bias, which I came from this, this hedge fund that was very fundamentally driven or thinking about companies in a fundamental way. It’s the same thing with data. Like we think fundamentally about the data. So if we don’t understand why something is happening, we won’t do it. And this is honestly, this is a big contrasting point to how the quant funds operate. Like for them, they don’t need to find real world causal mechanisms between the cause and effect. They just found a pattern. And they’ve seen that in the past, that pattern tends to hold true in these instances. And because they’re working on such like high frequency time scales and across like so many different securities, they just need to be right 51% of time. They’re making like 1000 millions of these kinds of transactions on a regular basis. And so if you’re a little bit better than 50%, you can make a ton of money. Like we are, we are much more concentrated, right? Like when we think about these problems, like it’s also an analogous to like the shotgun approach, we’re just like spraying out these pellets everywhere and they’re hoping someone we’re going to hit your target versus like we’re taking rifle shots. And our rifle shots have to be really accurate because we only get one shot on the target. And so when you have that, you have to have some version of this like causal mechanism of why something works. So to give you a concrete example, look at COVID, right? So the models that work pre COVID in whatever industry, let’s say, I know you’re in the travel space. So in the travel world, we had models that were forecasting hotel occupancy and revenues a year in advance. And those relation, that was based off of historical data because we knew what was happening based on the past whatever five, 10 years that we had data for, and we’re making forecasts for the next year. And those forecasts were awesome. They’re really, really good. But then COVID happens. And now, obviously, like something has changed in the real world. And so one of the things that you can do is to just say, okay, well, I can choose to continue using my old models, but those relationships are clearly no longer really working. Our accuracies have gone down. I can I can change a model and just say, okay, like what, how, but how do I change the model to account for this? So you can do things like you can create models that take into account what’s called a regime ship. So we had some version of history where this pattern was true. And we now are in a different regime where some other patterns might be true. But these the choices in these like to choose to switch to a regime ship model are driven by some something that happened in the real world. The problem with that is like, go back a year. And if I did not know COVID was going to happen, or didn’t know the extent of COVID, I will not have known to make that that change in my models back then. So in some ways, by using this this recent history, you’re cheating because you would not have access to this information before. And another example that that’s really relevant here is that, like I mentioned, we’re using credit card data to calculate the revenues for all sorts of companies. What’s happened post COVID is that so what what the result was is that the data has become much more representative of what’s actually happening in these companies. So in some ways, our models are becoming less useful, because I mean, our models are making all sorts of adjustments, and they’re still doing a bunch of that. But the data itself has become more more representative of what’s happening in the real world. And the question is, okay, like, do I need to change my model again? What’s happening here? If you go back to causal mechanisms, and you can start hypothesizing about things that are playing a role in this, one major one is that people are are much less likely to use cash in transactions. So cash was a big blind spot that we just didn’t see this was a group of customers whose behavior was not represented in our dataset. And depending on the company, that group of customers could make a huge difference. And now as those customers are moving to credit cards and moving to online spending, we’re now seeing a more complete picture, a more representative picture of all the customers of this company. And so, like, that that sounds like a plausible story, right? Like, you know, it makes sense to me, it makes sense to the people we’re telling it to. But in our world, like, it’s still just a story. So I don’t want to change my model just on, like, it’s good to have this kind of a story. But then the next step is to say, okay, can we actually validate this? Can we see and we do actually see this in the data, we can see how people how much how many withdrawals are taken from an ATM. So we don’t know where they’re spending the cash. But we could see that some people are used to be much bigger cash users than others. So do we see those people now moving on to the credit cards and so on. So the idea is that you once you have these causal mechanisms of what’s happening in the real world, you can inform model decisions. And then you test those model decisions. And if that ends up playing out, and it’s like, yes, cash is an impact, we now have a proxy, it’s not perfect, but it’s a proxy of cash usage. So we can now account for that in our models, and our models have improved versus just saying, Oh, our models are now like, you know, machine learning, it’s black box, we don’t know why the models change, but we’ll just accept the fact that they’ve changed. So that’s been our bias is like, we want to understand what’s happening. And when we communicate to our clients, the majority of our clients are humans, right? So like, it’s not enough for me to tell you, Hey, my model just did this, like, trust in my model, like you have to understand the story. And, and the way to do that is through stories and through these explanations that make causal sense. And you’re like, Okay, yes, this makes sense. This adjustment makes sense. I’m now going to trust this new model. Yeah, I find that’s a real big problem in selling models, right? Because there, as you said, essentially black box, and it is a heresy in the machine learning community to ask these questions, you just explored, right? You, you cannot make, there is no hypothesis, there is no causal relationship, there is never a question why this model works, it works or it doesn’t, right? And then that’s called the loss function. It’s it’s inside of it. And I find this on one hand, obviously, it creates is a normal scalability because you never have to worry about these difficult questions. On the other hand, we are very often predicting the past. And we have, as you said, when major assumptions change, the models just go awry, it is not much you can do about it, you just have to wait until you have enough of a data stream, you cut off the data, and then you start from scratch again. And then you just basically ignore anything that was beyond that data stream started. When you look at really cool data sets that we don’t know about, like, I didn’t know you can just order custom satellite imagery just like that, I thought you need to be like, you know, the CIA or the Russians, what are really cool data sets when when you, I don’t know, maybe your family or other friends, when you tell them about this, they say, well, really, that’s possible, that’s relatively low cost, say under $10,000. Yeah, that’s a great question. I will say that this people used to be much more surprised when we first started this, you know, like five, ten years ago. And exactly the point like we have been, we’ve worked with satellite imagery and it’s exactly you say you can reposition satellites, you can take the images from the satellite, because the satellites are constantly flying over the planet. And you can take what’s given to you, but you can also pay extra and you can reposition the satellite to get special images for you. And it does, it is very science fictiony, like this ability to go in there and try to collect all this kind of information. So just one point on the satellites before I move on to some of the other categories that I think are interesting. Satellites are definitely cool, like it’s really cool to be able to pull up that image and to have that ability at your fingertips on your laptop from a cafe in some random country in the world to just say, I’m now going to reposition a satellite. It’s not quite like that because you basically have to send the invoice to pay a bill and someone else does it for you, but it’s effectively where you go. The reality of it though is that once you get past this like coolness factor is that there are some major limitations with satellite data. One of the main ones, and it depends on the use case, right? So you, we mentioned the use case of seeing whether a factory is operational or not. That’s kind of a binary signal. Like yes, there are people there or like there’s nobody there. And that’s a very easy signal to detect from a static image. The problem with satellite imagery is that from a temporal perspective, they are very sparse. So the satellite might image the same portion of the globe, the same area, once every few months. And so one of the other use cases that people were using were trying to use satellites from the beginning was to monitor the number of cars in the parking lot at a store. And so, okay, yes, theoretically that would be, that’s a good proxy of the kind of volume that the store is doing. The problem is, if I tell you that in reality, you’re seeing an image from like a Tuesday in January and the next one is on a Sunday in April, perhaps different times of the day. There’s not much you can, you can do from it because obviously weekends are a much, play a much bigger role in the traffic to mall than does, you know, this, this image that you have. And so that’s one of the main problems. Like we don’t have regular, like daily, same time day images of the same parking lots over time. So, so the satellite, the satellite data has not been as useful for those kinds of questions. And it can be useful to like, do things like monitor construction progress. So if I’m, if there’s some big power plant, or if there’s a, some kind of project that I don’t have easy access to, because the middle of nowhere, there are barely any roads that lead up to it, it’s gated off. And I want to know, are these guys on track that to build their facility or their, their power plant, then yeah, using satellite imagery is awesome. But then like putting, putting yourself in sort of like in our shoes and playing the detective here, it’s, it’s cool because you kind of feel like a, like a secret agent, but the same time you don’t have any of the resources of the, of the government, right? It’s not like we can just do anything that we want. And so we’re like, okay, what’s the, what’s the poor man’s version of this? So I guess satellites would be super expensive to, and it’s physically impossible to get this data on a, on a daily basis. What else can we do? So then you start thinking, okay, what’s the next best thing if I want imagery? Well, can I pay some guy to like fly an aircraft over this, this area and take pictures for me? Like, okay, that sounds plausible. How much does that cost? And by the way, this is like, this is my favorite part about our job is that, unlike the textbook examples of like data analysis, we were just given a data set and it’s like, okay, implement some cool models on it. We were the emphasis is just on the models and like, you know, whatever the data is super clean. For us, like the data can be anything. And we tell the folks that, that join our company, it’s like, don’t be bottlenecked by what you, by just what’s handed to you, like use your creativity and figure this stuff out. So it’s like, yeah, like airplanes fly, we know that you can take pictures from airplanes, great. Okay, how much does that cost? I have no idea. Let’s just call some, like, how do you even start approaching that problem, right? Just go to a small airport and call them up. There’s like people that fly small aircraft and be like, dude, you’re flying, like, how much would I have to pay you? Now you’re talking real super, like James Bond type, right? Secret agent. Exactly. Exactly. James Bond, when he’s in trouble, and no one wants to help him, you know, and you’re just like, I don’t know, I got to go make friends and I obviously need to pay some money for this, but I don’t have a lot of money. And so how do we, how do we solve it? And yeah, so we can definitely do that. You can pay people and turns out it’s like for three grand, you can, you can get somebody to fly aircraft for you and take pictures. You can also pay people with drones, right? There are now drones everywhere. So you can pay a drone operator to who lives in that area. That’s even cheaper. And you can get videos, you can get better resolution stuff. You can also just pay people to drive by and take pictures from their car. Like if they’re on public property or public rows or sidewalks and you can just take pictures of these things, that’s great. Why not, why not do that? So, so there are all these different ways of collecting, collecting information once you understand what the, what the real problem is. But in terms of other data sets out there, I mean, like it’s, there’s a lot. I mean, there’s a lot of information that’s being collected. Like one of my other favorite ones were companies like antivirus companies that are, let me take a step back, anything that’s free. And this has always been a joke. Like if you’re not paying for something, you’re the product, right? So there are, they’re antivirus companies that were providing the software for free, but in exchange, they’re basically collecting all this data and selling it to people like me that, and we’re using it to identify people’s like browsing behavior and understand like the websites that they’re going to. You have data on everything from like mining operations to railroad companies. Location data is another category that we mentioned. So we’re all carrying tracking devices in our pockets, right? Our cell phones are constantly tracking our, our location, there are a bunch of apps that have access to that. And that information is increasingly becoming available for, for sale. So you can, yeah, I can, because that’s another example, going back to the mall example is like, well, why don’t I track the cards if I can track the people. And now I see them much more. I see them on a regular basis. I can see exactly where they’re going. And there are, there are tons like honestly, anything, any company that you can think of that has, that does something, they’re probably collecting data, and that data might be, might be useful. So, yeah, there are similar things across the world. Like there are companies that are doing this across the world. So if you wanted location data in Brazil, like it’s, it’s exists and in Asia, all these things exist. And many other also some more bespoke things that are relevant to some esoteric investment that you care about, like understanding data center consumption. One, that’s one of the, one of the trends we’re trying to figure out how many people are, how many clients is this data center have. And you’re like, Hey, how do I solve that problem? And you know, brainstorm a couple of the bunch of ideas. One of the things that’s really interesting is that it’s, you’re trying to think about what’s correlated to the thing that I’m trying to study. So maybe I can get access to like, you know, a number of people coming into that data center, but that’s not going to be representative of how many customers they have. One of the things that is, is electricity usage. So if I know how much electricity usage something is taken in, I can figure out how many servers they have in that data center. So then you ask yourself, okay, how do I measure electricity? And you have come up with like five different ways that you might be able to do this. Some of them are really crazy. Some of them are less crazy. Like one, like one of the things that we did two years ago, we’re like, okay, we should be able to measure electricity. Maybe we can get the meters, but if the meter is on the property of the data center, like won’t be allowed in and we’re not, we’re not trying to break any laws. But electricity travels through wires. And so we can see the wires going into this facility from a public road. Can we figure out how much electricity is going through a wire? And we’re like, how do we do that? And we’re like, okay, well, and this is where it helps to kind of be, to just be a jack of all trades, know a little bit about a lot of stuff, but definitely not enough that you can do any of these things on your own. We’re like, okay, the electricity has something to do with magnetism. Like, can we just figure out, can we put a bunch of magnets around the electricity pole and figure out how much electricity is going through those wires? And it turns out we actually found some academics that had done this kind of research and had built some tools to do something similar for very different purposes. We’re like, okay, maybe we can repurpose some of that to solve this kind of a problem. And so there are tons of examples. And the reason like that I’m, you know, I’m sharing these is that it’s, it’s, there’s just a broad range. And the only limitation is like what you can imagine and obviously, you know, the law because we don’t want to do anything illegal. I think it’s super fascinating. I didn’t know you guys are such agents. You’re like the Mossad in domestic Mossad. I think that’s, that’s pretty, I’m mesmerized. I didn’t expect that. And in terms of data collection, when I look at big data sets, I’m really astonished. People that track airplanes, right, flight stats and fly to where I always wonder how they did it. And it seems it’s these, this data is basically public because airplanes have these transponders. And all you need is a certain coverage of antennas. So they send out antennas to people for free with a certain agreement that they have to share that data once they collected from that antenna. And it is actually that expensive. Maybe it’s a million dollars, maybe half a million dollars, but a global coverage of any airplane that flies, which is very useful data, was really cheap with that model that they went for. And I think Cloudflare, you know, a big service provider for a content management system, they did something similar. They literally went to data centers, talked them into a sweet deal, just sent them, they never, they know, actually traveled or did any installation to just send them a box. And then that was all the installation they had to do. And then, you know, now they have all the data about web traffic, that’s, they give it away for free. So they must make a lot of money from selling that data. I hope they still producing about two or three hundred million dollars of losses every year. But someone is maybe with a certain kickback scheme in the future, really interested in that data. When you think especially of web data, we all hopefully have by now seen the social dilemma that documentary and Netflix that kind of makes the impression that we all just as puppets, this marionette that Facebook and Google know so much about us, they can literally predict our next action better than, than we do. And I had Blaze on and he runs the Ripper and he says, well, we do have this data, but it’s all siloed out. We can’t, there’s so many dimensions to these forecasts. So yes, we are working on this. And, you know, it makes a lot, a lot of money. But generally, the models that we use, they are like five dimensions, 10 dimensions. We can’t model you so well, because we don’t have a thousand dimensions or two thousand dimensions. What do you think is actually going on with Google and Facebook? Are they so well, well integrated now with all the data to get from all the different sources? And I know they’ve been one of the biggest buyers of data that they can make really good predictions of what we’re going to do next. And we all have these scary moments, right? When we set something to Alexa, and then the next day we got these really creepy ads. Yeah, yeah. So I have a couple of comments to that. So on one hand, these models are, you know, they clearly, they’re spending tons of money. There’s a lot of, a lot of smart people that are trying to figure this out. On the other hand, it’s such a hard problem, such a hard problem. And to actually do the things and have that kind of control and the kind of predictive power on an individual level is we’re nowhere near that at the moment. And just even from the things that we’re doing ourselves on this, it’s like, I wish I could do that. Like if all the scary things are being portrayed in the media, I was like, oh, you can predict my next action. I can’t even predict my own next action, right? Like there’s so much, there’s so much noise. And it’s such a such a difficult problem that if anybody thinks like, I would hire anybody who can come to me and just say like, oh yeah, these companies are doing it. It’s like so easy. I want to do it. Like I would pay you a lot of money to come replicate that for us. And so that’s one thing. It’s like these things are constantly making mistakes. They’re constantly, they’re constantly not optimized enough to be able to do these things on their own. The only thing I’ll say is that the problems that have been able to have been solved and are really like impressive looking are very constrained. So you think about, you know, the the the algos that are playing, you know, chess, they’re playing go, they’re playing these things that are and even go like the evolution from chess to go, they’re making a big deal in the media, rightfully so, which goes a much harder game than chess, the number of possible moves or orders of magnitude larger than the moves in chess. But it still is a very, very, very restrained problem. So you have like these these restraints, you can only move in a couple of directions or certain rules that that govern the game. And then just a matter of like running a ton of iterations through that, which computers are really good at. It’s the same thing with with all these other fields, right? Like to talk about like Google and Facebook, like, they’re just advertising companies, they’re glorified advertising companies. Yes, they’ve gotten really, really good at figuring out what you’re going to buy and like in getting you some kind of an ad that you’re then going to convert on. And by the way, like even that, that sounds like that is their only business and these are the massive like tech companies. It’s the only thing they do, the only thing they really care about, right? That’s how they make money. And even then they kind of suck at it, right? There are all these stories with COVID when I think it was Uber, when these companies shut down their advertising on on social media, because they just, you know, they weren’t operating. And it made no difference that they were shutting down like 100 millions of dollars on these platforms. And it was making no difference. And obviously Facebook and Google, they have these like attribution algorithms, but they’re super incentivized to tell you, like, yes, this one ad generated like a bazillion dollars for you. And I know this from some of the corporates that we’re working with, by the way, like we, we ran Facebook campaigns and Facebook. And this was like a fantastic example that could not be true. The there was a promotion like a Black Friday sale, the day before the sale started, there was an email that was sent out to an email list. So only people on that email list got access to coupon code and they were able to buy. So we know for a fact that this on that day, it was all email marketing. And then there were there was, you know, like then we turn on the social media promotions and so on and so on. And so Facebook was taking credit for 100%. I’m not joking 100% of the sales that were happening on the day before you even showed someone Facebook ad. Why? It’s well because the way that they do their algorithms is that they take credit for like, there’s a window of time that they take credit for. So if you saw an ad now, and you bought something later in the future, they’re going to say, Oh, it was because of my ad. And there’s some truth that right. But then, but in this case, it was so obvious that this stuff was like, not even we’re even showing them ads the day before, there may have been some like legacy things that over the past, whatever, before the sale started, they were seeing some of these ads, but it was like so clear to me that that was that was not true. So the point is that even in that example, where it’s super, a super tight problem or like, I’m just going to try to figure out like what ad correlates to what product, there’s still a lot of money that gets wasted. Because these ads, if you look at the conversion rates and how much companies are paying for their ads to convert, it’s not it’s not one to one, it’s not like you’re, it’s not like they’ve gotten it so good that you spend you’re making your printing money with every every ad that you’re taking out. And that is if that’s not a compelling argument as to why machine learning and AI kind of still sucks, I don’t know what is, right? Like they’re spending all this money, yes, they’ve gotten a lot better at it, but they’re still pretty terrible in the grand scheme of things. It’s better than the TV advertising and so on, let alone we’re trying to extrapolate and now say, oh, these companies are now going to know X, Y and Z about me that has very little to do with with advertising. So it’s a really, really hard problem. And it’s also, these are the kinds of problems that are fun to work with, but we’re definitely not there yet. This isn’t the kind of dystopia where these big tech firms know everything about you and they’re doing all these terrible things with it. It seems like looking at it from the outside because they reach such a wide swath of the population. So imagine an Uber ad, I’ve seen it probably 50 times by now and I’ve been a user for so long, I don’t know why they still show me the ad, right? I don’t get it. And what else can I do? I already give them my money. But people feel that they are in that business of accumulating the data, they just don’t know what to do with it yet, right? So you’re kind of in the opposite problem of you were with the data center where you knew the problem that you wanted to solve, you just didn’t have that data, but they get this massive amount of data streams. They don’t know how to make use of them in a proper way. I agree with you. That’s certainly our fears are ahead of what’s real right now in the more science fiction, but it’s easy to see if you can see every single email, if you can analyze the email in a way, in a cognition way that another human would do it, right? If you are the detective and you say you want data on a specific person, right? So then I understand that’s rarely the case, but you would want access to the email, you would want access to the browsing logs, you would want access to when they do what, at what geolocation, you, all this data that we offer them for free, it’s, they already have it in their hands now. They might not make perfect, perfect use of it, but I, if I want to abduct someone, this is the kind of information I want. I want to gobble up all this information, know all the routines so I can like surgically pinpoint my abduction plan and we’ll go to plan because, you know, people have habits, they don’t, they don’t do, they don’t behave randomly. And maybe they’re not there yet, but maybe it’s only 10 years from now, 15 years from now. It’s just, it seems to take only better computers and bigger models and more algorithms and a new GPT five. And then you’re there because the data is being given to them on a, on a silver platter. I think, yeah, I think that’s, that’s, that’s all true. I will say that the other component to this before making all this stuff possible is for better or worse is you’re still humans and human organization is hard. I’ve seen this, I’ve learned much more about it now that I started my own company and it’s, it’s, you know, it’s, it’s kind of funny because even though I started the company, I run the company, I’m still on a daily basis. Like the joke is like, I wish I was a CEO of this company because like people don’t do what you want them to. It’s, it’s, you can’t, it depends on your style, but you, you’re not this like authoritarian leader that can impose their will through fear on their people. You still have to like collaborate, you have to incentivize people, you have to design all these kinds of mechanisms. And over time, what happens with big companies is that there’s this inevitable slide towards bureaucracy and there’s more complexity, more silos, different groups are finding it hard to communicate with each other. I’ll give you a fun example of this. I was actually just talking to someone recently who was telling me, so I mentioned the, the, the credit card data that we’re using, right? These are a handle of about, let’s say like one or two percent of the transactions out there. It comes from a couple of like banks throughout the country. And the stories I got, one of my friends was talking to someone at that bank that was working in a data science capacity. And they were talking about like, like the person at the bank was like, oh, they’re trying to answer these kinds of questions. And then my friend was like, why don’t you just use your, your credit card data that you guys have access to. And, and the guys like, oh, there’s, I can’t access that. There’s all this like legal stuff that we just don’t have. We don’t have access to it. And he’s like, oh, it’s funny because I do. And it was true. Like we literally had access to his banks credit card data through this panel that we’re purchasing completely legally through like everything was permission. Everything was, was totally kosher, but the people internally had no idea this even existed. And they were just like, they’re like blown away that this, this kind of stuff happens. I think the point is that, yes, like the technology can get through the data can get there, but you will still have these issues of, of how do you structure a corporation? How do you structure the right kinds of incentives? Like what is the incentive for someone to actually like, why do they need access to this data? What are we going to do with it? What are the questions they’re looking to answer? And I think to kind of take a step back here, I think it’s important to talk about some of the privacy and the legal concerns around all this. And it’s, there’s a, you can paint a very scary picture about all the things that can happen. And one of the things that I think from our standpoint, which is for the first time, probably ever, you can say that finance, the finance industry is one of the more righteous players in this space because, and this is going back to our, you know, stereotypical like sociopathic tendencies. Like we don’t care about people. Like we don’t care about you as an individual. I’m not using this data to spy on you. I’m using the data to spy on a company. So individuals are just annoyances that we then have to aggregate in our ultimate source to try to figure out what’s happening with, with these companies. We’re not trying to get you to buy our product. We’re not trying to get you to vote for our candidate. It’s all just like we’re trying to figure out what is actually happening. We are observers in the real world trying to figure out what’s going on to the common denominator between Wall Street and communism. Exactly. Exactly. Yeah. Yes. I’m joking. Go. Sorry about that. No, no, no. There’s definitely, yeah, there’s a lot of like funny things about that. Actually, the like some of the, I’ve actually been thinking a lot about this. I was originally born in Romania when it was communist. And so I grew up under the Soviet, you know, like Iron Curtain. And going back, I mean, there’s whatever, not to get political, but like looking, it was, it was pretty terrible. I mean, it was terrible what was happening there. And, but now like looking back at it and looking at some of the things that they were trying to do, it’s super interesting, right? Because they were trying to, to plan the economy, like plan the economy. That was the whole concept behind it, except that they didn’t have enough data to do this on a good level. So there’s like some part of my brain that wonders whether if they had access, the kind of data and computing power that we had today, that you can potentially like plan, you can definitely plan it much better than they were able to do it then, probably not as well as, as just the, you know, the free market ecosystem does. But at the same time, it’s not like our free markets are totally efficient. There’s tons of inefficiencies in the organization of it. So you can imagine some benevolent communist algorithm of the future that decides like, hey, I know what’s, what’s better for each of each of you than you do yourselves and then starts making decisions accordingly. So I think, you know, there is something to be said about these. And it’s something like that. One of my other friends said is that there’s no shortage of confidence in this world. So everyone thinks that they can build this one centralized, like be all and all that’s going to coordinate all of human activity. And yeah, the rally, maybe at some point we’ll have the, you know, we’ll have the singularity, we’ll have the ability to do this. But for right now, it’s still, it’s still very hard. And again, it’s ultimately because of these, these people issues. And that’s, that’s something I tell clients or people that we’re talking to about this, when they ask like, what’s, you know, like the biggest challenge of working with data, and the biggest challenge is people. Like it is, like, yes, it’s hard to work with data. Algorithms are hard. There’s so many problems with the biggest challenge are the people. Like how do you, once you have this information, this insight, how do you effectively communicate it to someone? How do you get someone? Like if I’m trying to convince, not convince you, if I’m trying to, you’ve asked me a question and I’m presenting you with an answer based on, on this data, and you don’t agree with that answer. What happens? What do you do in that scenario? Most people, and this is even the people that know they shouldn’t be doing this, but at the very least, if they don’t agree with something, if they agree with it, they’ll take it and it’s fine. They’ll take it, they’ll show their boss, they’ll do, you know, put on an investment, they live happily ever after. If you don’t agree with it, the first thing, even if you’re trying to be intellectually honest, is to say, can you double check that? Or have you thought about this? Or have you thought about that? Which are all very fair questions. And like oftentimes we do end up finding more things if we dive deeper. But would you have asked me to go deeper if the answer happened to agree with you? You know, and if it was showing you exactly what you expected, would you have still asked me to dive deeper? Because dots of a mistake are still there. So there’s this element of like… Real truth is in antagonism, as the Greeks said, right? So if you are not… That challenges you and that typically leads to better insight, because you’re forced to innovate, because people are, you know, antagonistic to you, and you better find a way to persuade them. And if you have to come up with new arguments or new ways of thinking, that at least is the onset of innovation. That doesn’t always happen. Sometimes it’s just not possible. You can’t convince anyone, everyone. But you usually find that in a certain amount of conflict, in the civil conflict, right? If we start hitting each other and we start being violent, then there’s no use to it. I think that’s what we owe to the Greeks, this insight. Maybe they took it from someone else, the Babylonians, by the way. I think that, yeah, that’s a very, very good point. And yeah, there was a book I read a few years ago called, it’s by Annie Dukas, about poker. And she’s a poker player, and she wrote all these lessons that she’s learned from poker. And this is a couple of her podcasts, yeah. Yeah, she’s great. I think she has a new book out. But one of the insights was exactly that, is that you are often, as much as you read, and this is like, by the way, it’s like an even interesting corollary to this, which is that the more educated you are in this space, the more blind you become to your weaknesses. So the best way to see yourself in a mirror is to have other people antagonizing you, right? So people challenging your ideas, because only through someone else’s eyes can you really be objective, no matter how much you try on your own. And a great example of this is that we have clients that are just people that we’ve known through this, through our work, that are contrarian investors. And the idea of being that you have, because if you’re going with the herd, it’s often hard to make money. I mean, you’re just basically hoping that the tide rises all boats. But to make a lot of money, you have to believe something that no one else does, right? That’s the pure deal question, like what’s one strong held belief in the world that you disagree with. But that on its own can be a bias, right? If you’re someone who always looks at the downside of everything, and you think you’re being antagonistic and you are, right? But that on its own can end up being a bad thing, because that’s the only lens you’re seeing the world through. And you can just say, hey, wait a minute, and this was the funny thing with the fund where I used to work, like we invested a lot in distressed investments. So everything was like companies that were doing poorly that were whatever, hopefully going to turn around through something or not if we’re shorting them. And so everything was a distressed investment. Like the whole, you had these like terrible views about the world, like very pessimistic views about the world, because that’s what you’re trained to look. So I think, yeah, it is a hard problem to ultimately be objective as a human. And yeah, like you can invest, you can probably do is rely on others to point out your weaknesses. Yeah, it’s this very interesting crowd. And I absolutely admire them. Often macro investors, value investors who have a very contrarian view on the world, we all believe in this, you know, the world is going to be a better place in 10 years from now, more or less, you believe in this. And it’s usually true, right? So it’s useful, I think, as a self fulfilling prophecy as an illusion, almost. And we all kind of as a herd, we go towards there. But we know there’s this frequent crashes in between, we know there’s certain things that never take off. It’s, it’s very refreshing to hear from such contrarian investors. But I think they will always be a small minority of the of the complete picture, but they’re very useful. I think this is like people on the left and on the right, there’s all these extremes. And they’re also necessary. Because without these extremes, we would never find out where is the middle and where could we come together. So there’s this, this, this white spectrum that’s absolutely necessary. And there were obviously people were somewhat concerned that social media will run at the algorithms, especially they will run into this one box where they say, okay, this is this is the established opinion. This is the herd mentality. So to speak, but in social media, because in place told me that often the algorithms are just not smart enough. So anything that doesn’t go along that line gets gets flagged. And then because a lot of the the manual reviews, they’re scared to know what to do, they said somewhere in Bangladesh, they’re like, okay, just throw that account out. You know, we don’t need that opinion here, because it’s there’s no effect on our revenue on our bottom line to have this account or not, even if it’s a big account. And so a lot of absolutely necessary and good inputs, not on its own, but like for the wider picture, inputs in social media got thrown off board, which I think is terrible for the society. But obviously for these companies, they didn’t have to, they didn’t even think about that. And I think Zuckerberg then gave that speech. And since then, it’s gotten a little better, I feel. Yeah, yeah. And I think that’s a very good point. Like the way that the way that I found it helpful to think about about what we were just talking about is there’s a difference between forecasting something, right? Well, we have a concrete question, and you’re trying to figure out the answer to that question. That’s like, that’s an easy thing. But what ends up being confounded with that problem is what are the questions to begin with? And these are actually like two separate dimensions in your in your old diagram, where somebody can be really good at coming up with very interesting and crazy scenarios and questions to ask. And this is again, going back like Phil Tetlock and the work that he and his colleagues have done around this, where I think they call it foresight, as opposed to forecasting. So foresight is somebody who can come up with the right callus, the right driver of something happening in an environment. And we see this all the time, like where there’s a certain scenario that we just never like, I don’t know, just taking on some things in the recent past, like Russia invading Ukraine. Like would you have thought that was a likely possibility in this day and age? Most people would have never even probably considered that as a possibility. And there are tons of these are people who are really good at coming up with these like crazy things that you never think of. At the same time, those people are not necessarily the ones to assign the best probability for those because those people tend to be people that are always paranoid and they’re always thinking about the craziest things out there on on the negative side, but also on the positive side, right? There are these like permanent utopians that are thinking everything’s going to be amazing. Like, you know, Elon Musk and others are like, oh, we’re going to go to Mars, we’re going to colonize space, we’re going to do all these crazy things. Like, yeah, sure, like we most people would have not really thought about that in a serious concept. But again, those people might not necessarily be in Elon in particular has not been great at getting the timing right of his predictions, right? So coming up with an actual probability of this is going to happen within this timeframe is not necessarily correlated to people coming up with these kinds of ideas. And yet we need both. And when you’re when you do see both these things, you see like an interesting question and a forecast behind it. To your point, it’s like, it is, you have to think about this from like a Bayesian statistics framework, which basically boils down to there’s a prior there’s a baseline probability of certain things happening in the world. And you should always start with that. So how often do wars happen between countries? How often do whatever like any sort of whatever the event is that you’re trying to predict, how just seeing to extent possible, how often does that thing happen in the past? And then going back to example of the contrary and investors, it’s like, yes, like there are constantly like accounting scandals and frauds and all these things that are terrible and trying to identify companies to the short. But you should be aware of what is the baseline like out of 100 companies in a two year period or one year period, because most of these investments, you know, you don’t have that much time out of 100 companies in one year period, how many of them end up being exposed for being accounting products? And starting with that. And so if that baseline is really low, maybe it’s like one out of 100 that in any given year has an accounting fraud, then it’s saying, okay, like even if I think this is really, really likely, like in this one case that I’m investing in right now, it’s super likely, maybe I go from 1% to like 5%, maybe 10%, because I append all this additional evidence on top of my baseline, and I bring up that forecast. But it’s very different if you’re not starting with the forecast, you’re presented with like, here’s all this evidence why this firm is a fraud. And you’re like, oh, holy shit, there’s like a 90% chance these guys are fraud. But you don’t, you forget about the, you know, again, the baseline, we’re supposed to anchor you to the reality of what’s happening. And so I think that’s the point that you’re making is that things have gotten better for us as a species over the past couple of centuries, right? Like pretty much for a very long time, things have been, have been getting better. And yes, there’ll be ups and downs, there’ll be certain things to be pessimistic about. But if you start with that kind of, that kind of a baseline, then you can end up being, you know, being much more accurate in your, in your ultimate forecast. And that’s, yeah, that’s something else that I think is really, really important because a lot of the discussion, especially in my world where you’re talking about data, you’re talking about all these things. And rightfully so, people are concerned about the privacy implications. And there’s tons of articles, right, about the invasion of privacy here and all this regulation, all these things that are popping up. However, it is, it is really easy to scare people. That’s just another piece of human nature, right? You can run your company by scaring the share of your employees, and then they’ll do what you say. But that tends to be, it’s a much more unstable equilibrium, because the moment you lose some amount of strength, or they just no longer want to follow you, you can end up blowing up. Again, I thought that’s, that’s only true in Wall Street. Yeah, well, yeah. Probably not anymore, probably not anymore. But, but maybe we can, I think we’re getting there. But I wanted to ask you on your opinion on the singularity, especially what other investors are thinking. And if there’s anything they already do to prepare for this. And by singularity, I mean, not that we have the all these intelligent robots walking around, it’s, we have access to, in about 2040 to a million brain, million brainpower, not real brains, but, but somewhat general artificial intelligence that we can basically, and similar to what you guys do right now, we can, we can ask a question, it will go out, find the answers, find the data for it, and we’ll transmit ancestors and certain abstraction that will give us one consolidated answer. And that will be really cheap for $1,000. We get a million of those mini AGI’s that will not be conscious, probably, maybe develop. Is this something that investors already forecast and the trade on is, or is a two science fiction? Well, so people, it is possible to trade on science fiction, but it is, it’s not terribly common. And you have to think about it in the context of going back to all this conversation we’ve had about timing. So most in timing, by the way, boils down to human incentives. And what are those incentives? Well, at the end of every year, you’re, if you’re a hedge fund, you’ve taken in money from all sorts of all sorts of other investors that call LPs or the limited partners, and they want to know how you did, right? And so if you don’t perform well, they’re not going to be happy with you. If you perform well, they’ll give you more, more money. It’s, it’s, it’s simple. It’s probably very flawed, but it’s just the way the world works. And so you have these very short term incentives. And then beyond that, you didn’t now have like those incentives cascade down, right? So if you’re an analyst at a hedge fund, you might be right in even like the two, three, five year horizon. But if you’re wrong, if you’re terribly wrong this year, you can get destroyed. And we see this all the time. And then this is the, I mean, we can talk a lot about this sort of like risk management and how do you, how can you be smart about incurring heavy losses in the short run in the pursuit of a much larger goal? And the reality is super hard, right? It’s really, really hard. Most companies cannot do that. And so if you end up having a terrible year or even most businesses don’t have the luxury of, of withstanding that. And so to invest in something that is, even if it’s like a long term trend, maybe it’s not even talking about the singularity, because that’s, you know, super who knows how far out it is, but it’s much more science. 2040 one day. It’s, it’s, it’s nailed down in 2038. Actually, in fact now, so it’s not that far out. Yeah, that is, yeah, well, that’s, that’s, that’s true. I was gonna say like, yeah, even things like self driving cars or some of these other plays that are, we’ve seen demonstrated evidence of their existence, right? People driving Teslas that have self driving features now that technologies roll out to all sorts of other cars. Like even that is, it’s hard to nail down exactly one like Elon thought that we’d have fleets of self driving cars by now and we haven’t. So the problem when it comes to investing is that you can be right in the direction of something, but if you’re wrong on the timing of it, you can be screwed. Now there are definitely ways of doing this. I’m trading on it. So for example, venture capital funds, they have a much longer time horizons. They can wait, you know, five, some, some of them will say 10 years for something that, but it’s usually pretty rare before some, some event happens. And then you have some other like the product, the most long term money out there is through these very like more traditional institutions or family offices where this is intro generational wealth and they’re not interested in ups and downs over the next couple of years. They just want to know that their grandchildren are going to have enough money to survive. So I mean, that’s, that’s kind of a joke is there, you know, they’re, I’m sure they’re, they’re very well off if they have a family office. But having said, I mean, there’s definitely plenty of stories of, you know, rags to riches through the rags again in three generations. And so some of these investors are betting on the longer term environment, but it still hurts a lot when you, when you’re wrong, I mean, you get crushed. And that’s, that’s true in anything, whether it’s investing or anything else in life, it’s like the people who survive aren’t like, you know, big boxing is an example. You don’t have to be the strongest boxer to win. You just have to be able to take the most punches. So if you can get hit over and over and over and over again and not go down, like that’s entrepreneurship, right? Like that’s the reality of the majority of our human existence. And it’s really hard, especially when you have like other people depending on you, like I’m just speaking from, from experience, starting a company, like you can go years without seeing the results that you wanted. And again, there’s going into any of these endeavors, you’re super optimistic, everything’s, you know, awesome. But when the reality like, you know, it hits you over and over again, the only differentiating factor is like, yeah, just pick yourself back up and keep going and try not to, not to be discouraged. Yeah, that is, that is the way to make survival happen, right? To have a certain amount of maybe illusionary positivism that keeps you up and just you keep on walking. And that’s, that’s the way to survive. And I think this is the, the pioneering, the entrepreneurial spirit, which is strongest still in the US, I hope it’s going to come back a little and in economic production and in productivity growth. I feel like, and maybe that’s just me, but talking to so many people here on the podcast, I’ve been more and more drawn towards this prediction that AI will have a serious impact on our productivity to the better. Because we know that the price of computing is going down like crazy, and this hasn’t changed in a long time, and it will keep on going. There seems to be, I just got a new laptop, and these new, these new MacBooks are so much more efficient. And forever, there was not a lot going on with Intel chips, and now Apple made their own chip, and it probably doesn’t cost them anything. It’s probably a $30 thing for them. And it’s extremely fast. And so Moore’s law is back with the vengeance. So these, these, these mega trends, they’re going to culminate in something big. Is that really how we envision the singularity? Well, that’s a good question. But if any of those things are true, I feel like we will see a lot of productivity growth and in turn, GDP growth in mostly the developed countries, not the developing countries so much, because all the data signs are the people who take most advantage of building the algorithms, they are all here in more developed countries, especially in the US and in Israel, and China probably too. So when I believe my own hypothesis, and you should never believe your own propaganda, obviously, but it’s not propaganda, it’s just my hypothesis. I feel there is a strong mega cycle of tech productivity growth. And it happened for the internet. It maybe not as measurable, strangely enough, right? We seem to have gone down in productivity growth. But the hope obviously is that this easy decision finding and this, this, this knowledge finding will, will make a big impact on my turnout in a different way. But I wanted to ask you, when, when you’re knowing what you know right now, what would you invest in? Might that be industry? Might that be specific investments or ETFs? You know so much about data. You know so much about technology. Where would you put your money right now? Yeah, I mean, that’s a fair question. And just to give the usual disclaimer, that’s not investment advice. I mean, there are a number of so like, I mean, this is the honest answer that question is like, where am I investing my money? And the honest answer is I have my money and ETFs. So just like broad index based funds. Sure. Why is that? It’s for a number of reasons. And this is, I feel like this is the case with the decent amount of people that work in this, in this space is that the more you know about something, the more you realize how little you know. We spend our days and nights solving these kinds of problems. And we’re going so deep into some of these questions. And we’re barely still scratching the surface of what we’re doing. We have entire like, you know, entire company, entire teams that are working with us, our clients are thinking about these investment questions. And we’re putting so much, you know, blood, sweat, and tears into this investment that when it comes to terms like, let me do my own stuff, how can I compare it? How can I compete with some of these other people out there that are literally spending millions and millions of dollars that have some of the smartest people in the world thinking about these kinds of problems? So that’s the honest answer, right? It’s like, these are some very, very hard questions. And then another part of it too is that you can be, and this is a lot of this, you know, investment philosophy coming from Warren Buffett about how it’s, it’s better to find these whole things about finding value companies. But I think the more generalized version of that is to say that it’s really hard to bet on any specific companies or specific sectors, just because you that technology is going to, or some sector of the technology is going to do well. The reality is like, like, how do you first of all, how do you even measure that? Right? That’s, that’s another thing. It’s like, yes, if you’d have bet money on, on certain types of sectors or certain kinds of stocks that went up, that’s great. But you always have to compare that to the rest of market, just because if everything else was up twice as much than what you were invested in, that still doesn’t mean you made a terribly great investment. And so the idea is, again, there’s, there’s a lot of complexity in there. But having said that, like some of the things that I personally am bullish on or optimistic about, and this was back to the conversation we had about, about technology. And I do agree that there’s a lot of reasons to be optimistic about technology. So data, let me focus on data, just because this is the sector that I know will probably the best. It has data, you can think of it as, as some kind of asset, people have compared it to like, you know, the data is the new oil, some kind of a commodity that you can then do something with. And there’s this entire phenomenon happening with blockchain and all the cool things that you can do off of just, you know, this one piece of technology, which is the blockchain. But that to me, it’s a very specific, narrow case. And honestly, like the things you can build on top of a blockchain are very limited. I mean, there’s tons by design, there’s so many different constraints in that, in that system. If you, if you abstract one level beyond that, like this is just another example of data, right? And there are data sets everywhere. There are industries, like I mentioned, every single company is collecting some amount of data. And those data sets are becoming digitized and becoming more accessible by others. And what we’ve done and what a bunch of the other companies in our space have done is that we build businesses on top of these data sets. And this is a real like growing segment of our, of our economy. And we’re obviously, you know, like the, the economy has moved over the past, you know, half a century more towards services in the US. To me, data, this is like the growth sector, talking about productivity changes. Like there’s so much information to be gained from this. We still, from a scientific perspective, only know a small percentage of like all the known truths out there about our own physical world, right? And especially when you go down to the social sciences, they’re much harder to understand and to predict people’s behaviors based on, on this kind of information. But it’s starting to become possible. If you’ve looked at any, like any of the ethnographic studies or just thinking like whatever it is that you’re interested in, right? Like if you’re interested in the study of humans and human social networks, like mating rituals, like all sorts of things that are from, that are nowhere near our world, right? These are like more academic, cultural things. We now have access to data that before you could never answer these kinds of questions, right? And there’s some, some hilarious, there’s one real book that I really like from the, one of the, one of the guys that, okay, Cupid, where he wrote about insights based on the data they were collecting from their dating website, right? And you’re learning stuff about our, something that’s so core to what we do that we’ve never known before. And, and so the point is that there’s just so many businesses that can spring on top of these, of these data sets. And, and one of the, one of the concerns is that if there is like, there’s so much focus on the negative parts of it, right? The, the, the privacy invasions and all, all these things which are, yes, like there are certain things that are scary about it. But let’s not miss the massive upside that it can be generated in terms of new, like businesses in so many sectors that can, that can spring up by just taking data sets. And there are literally companies that do this, that will take a data set and sell it. That’s all they’re doing. They’re taking a data set, maybe doing some adjustments to it, but oftentimes they’re just selling the raw data and they’re becoming sound like the gold rush, right? Like they’re just the brokers for shovels or the brokers for the, the pickaxes. And, and, and yet they’re contributing to the, you know, employment, contributing to all this, like not just like the overall well being of the country or the globe, but it’s also creating some like really interesting jobs. Like in my opinion, if you’re trying to like save, you know, manufacturing jobs or even, you know, manufacturing jobs are, can be extremely repetitive. You’re doing the same thing. You’re like an assembly worker on this line. Office jobs used to like, they’re honestly, they do not that much more different from like a mental stimulation perspective. There are lots of people that were just going in there. Even, even the people that like the higher paying jobs, you’re working on spreadsheets, you’re doing the same thing over and over again. You’re creating the same accounting reports. You’re creating the same, even in the legal profession, the same kind of like reviewing contracts. And I can’t tell you how many like accountants and lawyers are just like bored to death with their jobs because the same shit day after day versus the cool thing about data is it enables us to be creative, to ask, to be curious about, about the world and to have a tool and a mechanism by which not only can we express that curiosity, but we can do it in a way that is, you know, putting food on a table and it’s, it’s can potentially like, you know, positively impact the, the world. I’m fully with you. I feel like the, the emergence of data and obviously the, the availability of cheap data, right? And not everyone can afford data sets that are several hundred thousand dollars, but the ability to literally everyone to, to run the algorithms and their, their thought process, right? Literally just what questions can you ask? It’s the new, the new biggest problem. If you have the right question, you can channel that, that, that curiosity into getting data for free or write cheap. And they’re just running algorithms on it and put this result on wherever you want on a new GitHub, so to speak, right? I think there is so much in there and it goes from, from understanding our body, understanding every single cell. Once you have the data about every single cell, you can easily see what happens if we make some manipulation. We now just got to our DNA, which we can actually manipulate. We don’t really know what’s happening. We did, but we just had that last November, the protein folding results. So we, we, we’ve seen that in these simulations and that’s what our brain does literally, right? Abstract thinking is we simulate real world situations. Now we can simulate them out way faster. And if we can throw a million of these intelligent, somewhat intelligent solutions for the price of a thousand dollars at a problem, I don’t know what we can’t solve, right? We can solve nuclear fission. Maybe we can, we can, in a safe way, we, we can solve fusion. We can, all these things, space travel, all these things that would take a lot of money because we have to put scientists at it that have 40 years of education and they’re really difficult to, to hire and they’re different, they’re expensive. If we can just put a million AGI’s at it, maybe the results are pretty good. So I’m extremely bullish about this long term scenario. Right now, we really don’t know how it will work out. And there’s going to be tons of jobs that will be destroyed. And the question is, will new jobs come up at the same time? Probably not. But there’s going to be some delay. But as we’ve seen people coming out of the fields and everybody was worried, there’s no more food, right? When they went to the factories, but they turn out way more food for everyone. And everyone has a higher standard of living. And now we had the office jobs. And I don’t know what, where are we going to go next? Maybe I’ll sit at home and are lonely and have our head in the cloud. Maybe that’s the price to pay. But, you know, honestly, it’s, there’s a reason everyone just wanted to stay home with COVID. Yes, there is a virus, but on the other hand, I felt people realized, man, this is really comfortable. And I make the same, if not more money. And my living standard is drastically gone up because I suddenly are the boss of my own time. I can do whatever I want whenever I want with some restrictions. And the zoom poll is so much more convenient than commuting to an office for two hours. Yeah, I mean, so just a couple of reactions to that. I think one is we’re talking about this earlier about, you know, GDP growth that’s driven by productivity growth. And that, like just to kind of dissect that a little bit, especially in the context of COVID, that is, so in a lot of the things that we’re doing, we’re trying to figure out, we’re trying to dissect our own thinking. And a huge part of that is to dissect the assumptions that you’re making. One big assumption that underlies, like, all of economic theory is that GDP should keep going up, like GDP growth. I know in questions, like, why should GDP keep growing every single year? But if we do start questioning that, you end up with some really interesting, interesting hypotheses, right? It’s like, okay, so we want, we’re constantly working more, we’re constantly trying to produce more and more and more. And yes, transitioning from hunter gatherers to farmers to factory workers, that came with differences in the standard of living. But that is not just like anything else. Like, if we study math, a lot of stuff can go back into simple functional forms. This functional form, in my opinion, of human happiness, like, yes, there’s a period where increasing GDP growth is going to increase human happiness. But at some point, that’s going to taper up. And we’ve already seen that, right? Like, GDP has grown so much. And yet, we don’t, how much more stuff do we need? We now have access to, like, the whole trend now is tiny houses and minimalism, because we have too much crap everywhere. So it’s not like, yes, back in the day, I couldn’t speak more from experience when I grown up in a poor communist country. I have a daughter who was just born a month ago. And looking back at my baby pictures, which by the way, in black and white, looking at the toys I had in my crib, they legitimately scared my wife, who was American. And so we, yes, I’ve come a long way from having nothing and having just standing lines to divide bread and butter, to now being able to, like, order that on my phone and gets delivered to me. So, yes, there are certain steps that make a huge impact in your standard of living. But if I had, you know, 10 times more money than I have now, or 100 times more money than I have today, what would I really do? I mean, and it’s like, yes, there are certain things that you do, maybe I’ll do a little bit more travel, maybe I’d live in a bigger house, but they don’t even really need a bigger house. Like, I’ve been living in, that’s the one thing about, you know, like New York City that’s just living in there that makes you more comfortable with smaller spaces and making do with less. And so I think that’s one important aspect of this. And your point about COVID is that, yeah, we’ve been living in these, in our apartments, we have Netflix, we have, you know, we have family, we have the just ability to sleep more and to just chill a little bit, you know, not being on, especially like being in New York City, where it’s constant like rat race, you’re never, it will be embarrassing to tell somebody that you just didn’t do anything in the past weekend, you know, it’s like, you’re always have to be doing something involved in social organizations or what have you or like, obviously, everyone loves to say that they were working a lot over the weekend. And, and yeah, having a break from that is kind of great. So maybe we don’t need GDP to be increasing, especially as, you know, computers or these things are taking on some of our work, maybe we can focus on some of the things that we enjoy. I think a pretty funny example of this is we’re talking about the singularity. So Kurzweil, who wrote the book about the singularity is, you know, this thinking about all these like science, sciencey things, his daughter, her name is Amy, she’s a cartoonist, and she has cartoons in the New York, I should like own one of her cartoons, she does make fun of about a lot of these like, like robots and singularity type topics. And I think it’s a pretty example, like, you know, the daughter of the man who predicted that like AI is going to take over the world. So you know what, I’d rather focus on something more artistic and do something else with my time and try to advance all this technology stuff. So yeah, there’s plenty of opportunity for us to rethink about our priorities. And this is from just like wealth management, sort of like one on one, like what’s the best way to have more money? It’s to spend less, like that’s literally the easiest answer to that question. It’s much harder for you to double your income than it is to cut your spending down. And so yeah, like do we need all these things, like can we reshuffle our lives, reshuffle our priorities into things that are more important to us, then that could be another way to, you know, be happier. I can, I can, I can hear the European is strong in you. The Star Wars resemblance. I grew up in Europe too, also on the other side of the Iron Curtain. And I agree with what you just said. Yes, there is a limit to consumption in our current state of mind. And there is a limit to exhaustion. And there is, especially in America, we err on the side of doing too much and being too busy because we all, this is our favorite thing, like being an entrepreneur used to be the favorite thing. And now our favorite thing is to consume and it’s too, too marvel and materialistic things. Yes, these are all excesses. I’m fully video. But here’s the other side of that coin is whenever you look at the history of the world, whoever is able to be more productive, mostly that’s through innovation, right? So the, it’s not by working 100 hours instead of 50, that’s not being more productive. But if you use innovation to make, to produce the same output with less input, less energy or less time consumed, whatever that is, a less gold or less platinum, whatever is the scarce thing at that moment, that’s the, the group that has a chance of surviving and this won’t end. Now you can end it like, and that’s called socialism. That was and that the competition was ended and we all was this idea, we all make everyone give them their fair share and there’s no access, right? But it, and it sounds good and it probably works and it works with a certain group of people. I feel if you take scientists and I would, I’d call them the elite, they would be fine with this for quite some time, not forever, I feel, but for quite some time. But because what you kill is this, this motivational factor, the ability to, to look forward to a better future for yourself and for your children. And this might change, right? We might go through another phase and maybe we’re going to look more socialist and it’s, but there were good things to socialism, you know, but I, I thought it was horrible, but there were good things looking back, especially this, I have a different picture. And also when I go to countries that recently came out of socialism, that is a different picture than when you live under this regime, right? I was in Mozambique and that struck me as a pretty decent country compared to the rest of the neighborhood. But I don’t want to go through 30 years of Mozambique and Maoism, you know, that that was terrible enough, what happened in Germany. So I think this driver of this freedom from coercion, this ability to experiment this, but, but still have a rule of law and then focusing on productivity growth through innovation and, you know, onto entrepreneurs in the end to make it happen. We can innovate all time long, but if you don’t make it happen and put it into real world products, it doesn’t help much. If you do that, I think you’re fine and you’re going to meet the creator one day. Let’s put it in an old test them and context. If you don’t, you sooner or later get lost. You might still have a good life and you can live off the land. Say, we would hunt together or still do in Papua New Guinea, but I don’t know if this is living up to our potential. I feel what we are up to right now with all its flaws and all the reliable or vulnerable we are by relying on technology. I feel we are, we are on to something and maybe it’s the machine intelligence will take off and, you know, we’ve been losing track of this, but we’re definitely on to something. Yeah, look, I agree with you. I mean, I think it’s, I was even looking back at my own career to kind of dissect this a little bit. So when I was working, even when I was working at the hedge fund, there came a point where I legitimately like couldn’t stand my job anymore. And I know I was talking to other people that were working in and this was, it was, we were getting paid really well. Like everything was on from the outside seemed perfect. But on the inside, it’s like, for various reasons, there was just like so much is like the repetition of it. It was that the work that we were doing wasn’t, we weren’t seeing the impact of it. I’m not talking about like in some sort of like, you know, grand moral case of the impact, but literally, like, I want to see like, I did this report, I created this analysis, did anything happen with it, or even worse, if someone read it, like essentially threw it out and nothing changed as a result of that. Like that is morally crushing. And yet we were working a lot and we’re very busy. And it’s actually talking more about my friends. And it’s like, you know, can’t say that we were like lazy because we know was the exact opposite of it. And yet it somehow feels lazy, even though you’re working long hours versus when I started my own company and couldn’t be more opposite from so many regards, like, you know, I haven’t paid myself anything. It’s been five years since I’ve been running the company. So we’re doing this like this sort of like old school way where we’re trying to get customers to pay us where we haven’t raised any outside capital. So all the money that we’re getting in, I’m hiring people, I’m growing the company so we can grow the business. I’m obviously like lucky enough to be having able to do this because I had savings from before. And yet the working in this company has been so much more fulfilling because if you think about like growth, I agree with you that there’s something fundamental to human nature about growth, we want to grow. But what I posited is that it’s not just monetarily. It is what is much more important is to grow personally from an academic, I’ll give you a couple of examples of this. I had never sold anything in my life really before I started this. And that is the first thing that you have to learn how to do if you’re trying to go out there and, you know, eat what you kill, you have to go out and put yourself out there and try to sell something. And it comes off as super, it was for me very uncomfortable. Like if I have to, you know, ask you for money now, even though I believe in what I can do, I still, it is something that’s a little bit weird and then you have to have to get over it. And then not to mention getting better at it and improving on those things. And that’s just one element of what it is to sort of like map in it to be a more well rounded human. Like yes, I knew how to do how to be a nerd on a computer and how to do some of these analyses, but how do you convince people? How do you lead people? How do you negotiate with people? So you made a point earlier about people, other people want to get involved in data to try to find a free, like the fine free data sets and do something with it. Like yes, there are a ton of them and there’s actually a ton of public data out there that you can, you can monetize. And there are perfect examples of companies taking government data and because the government data is just presented in such a terrible format, they’re taking it, rearranging it, cleaning it up a little bit and selling it. And that’s all they’re doing. And it’s basically, you know, free on the data side, but it costs a lot in terms of labor. But there’s also another way, which is convince people to like to help you and work with you on something. So when we’re talking about these with these data sets, especially in the early days of our company, we were not a hedge fund, we couldn’t pay a million dollars for some of these data sets. And yet we were able to convince the data vendors to partner with us and share with us that data because we had something they didn’t, which was like, we were, we were the end users of it. We knew how valuable it was to us. And to your point, the hedge funds were very rarely giving them any feedback on their data. And so by collaborating and saying, Hey, like, I’ll work off of this, I’ll tell you something about data that you can then use for your own marketing purposes to sell it more. And that was like that to me is something that going, like, you know, just thinking about education, especially, you know, like for my daughter and others, that’s one of the things I wish I would have learned sooner. Like, yes, if there’s a focus on the academic side, like learning all the math, learning the programming, learning to do all these things, we should also learn how to persuade people how to find a common ground between people so that you can end up with data sets that cost millions of dollars that no one in their right mind would give it to some like, you know, two guys that they just met. And yet by forming the right kinds of like social networks by figuring like solving this kind of problem, you can become, you know, you can be a capitalist and get access to these kinds of data sets. And so, but yeah, and this all ties together. It’s like, all that stuff is awesome. And it’s super fun. And we’re learning a ton because I’ve never had to, like, I’ve never brokered multimillion dollar deals before on my own for stuff that I need in order to advance my own interests. And that is like much more important to me than like our profits at the end of the year, like having our business growing. It’s much more about what else are we learning? Do I know more now than I did, you know, a month ago or a year ago? Absolutely. Versus, you know, attacking some of my other friends that are still, you know, stuck on some of these jobs, they’re going to high paid and great. But they’re like, I’m still doing basically the same stuff that I was doing, you know, five years ago when you left. Yeah, I see you, you’ve taken this, let’s make the world a better place, really seriously. And that’s the right call, right? And I think we don’t do enough to instill that message in our children relatively early on. And kind of this is the only thing that matters now, right? It doesn’t really matter what you learn. It’s important that you motivate it enough to do this. And then there’s a couple of survival skills you still need, but we get less and less of those as life gets easier. It’s really using your creativity, finding that way where you feel like you want to make the world a better place, and then scaling up until you eventually really make the world a better place to come out of this simulation. And the way how we change that, I don’t know how they’re going to call this period, you know, we only know afterwards, but it’s this transition period that we have from the industrial age to this new age, and we call it digital, but probably that’s not it. It’s this very different world of what we are on our own. Now, this doesn’t mean we don’t collaborate, but we collaborate more through these channels in order to come up with better results and get even more specialized. Like the specialization that people can now put themselves into is off the charts, right? It’s something that maybe artists had before and to an extent it also people in Hollywood had that. For the rest of us, we never were able to be so extremely specialized and get away with it. It was just, there was no way. And as an entrepreneur, you can do this, but as you say, you have to learn how to sell. And if your community that you sell to, they’re all very conservative, then there isn’t much for you to eat because you will never sell anything, unless you label yourself as an artist. That was obviously an easy way out. And that’s a newfound superpower that people are just discovering right now. I think Silicon Valley is a little ahead, maybe has done this for the last 20 years, but has lost hope in between and now Google and Facebook have gone to the dark side. But I think there is this extremely positive, it’s almost like a brotherhood. I don’t know if that’s what you refer to, but it’s this brotherhood that enables you to A, be specialized, but also have access to the hive mind and download whatever you need. And it’s the fraternity that allows you to develop your own potential best. And I think this is this new world that people and still don’t have the right words, but it’s this new world that people are still trying to make sense of. And I think there’s a lot more coming in this development. Yeah. Yeah. I think that those are all great points. And it’s the concept of brotherhood is, I think it’s, yeah, it’s extremely important because none of this, none of the things that we’ve done, just personally or otherwise, could be possible without others, right? There are people who have different skill sets are naturally better at some things versus others. And by collaborating, by learning with each other, I think it’s, yeah, it’s possible to achieve different things. But it’s also interesting how all that’s going to change. We’ve always relied on others, right? Even from the early days, you had your tribe, then you, you have the concept of nations or nation states, at least like cities, you know, like different geographic units or social units that held people together. More recently, we have the company, right? Like the company is one of, one of the most, like underrated social groups of our time. And then, and it’s so obvious when you look at it, right? We spend the majority of our waking life surrounded by colleagues. We don’t even see our, it sounds like waking time, like our spouses as much as we see some, some coworkers. And, and yet it’s, and it’s okay, right? Because we end up like forming different kinds of bonds with them. But even that, that kind of an organization can, the assumptions behind it can be challenged. Like why are the people that are near me the best people to solve every single problem that I have for the next, you know, whatever five years I’m trying to do this endeavor. And that’s, that’s what this assumption you’re making. And then we started off this conversation talking about hedge funds and how secretive they are. That was the assumptions, like these are the smartest people that I can ever, that can bring around me. I formed my, my A team and we together are going to solve these problems. But the reality is that there are so many other smart people in competing companies in other industries. And, and so it’s like, how can we, how we know we need to collaborate with others? But what are some other forms of collaboration that might be possible? Now with COVID realize we can work with, with anybody, right? There’s obviously challenges and we’re seeing a ton of them, but there are, I think there’s still a lot that we can get from being physically close to others, but it’s also very possible that we have folks in Europe, in Canada, in Asia that are working with us on a regular basis to solve these kinds of issues. And like what I think is another direction that this is going to go to is to use more consultants, use more outsourcing, use some of the labor markets out there where you have, you know, sort of the gig economy that you can bring on people to do a specialized task for you and assemble a team just for this project. And then for this other project, we have a slightly different team. And we, we move on with, with that kind of a, that kind of a collaboration structure. So yeah, I think, I think fraternity is really important, but it’s, it’s also important to see how this fraternity keeps changing. And then there’s still some real big issues, is that okay, like now with, especially with COVID, there are people we have hired that have never met in person. And the old, the old school, you know, like fraternal organizations where, you know, the people in these organizations, even though they were not family, they were willing to die for each other. Like you have, you know, the military is a prime example of that, or able to create such a strong bond that I don’t, I honestly don’t think it’s possible to do that in a virtual setting, at least not with the kind of technology that we have now. So we have like there are certain things that our networks are wider, but the ties are somewhat weaker than, than in person. So I think these are some of the challenges that we’ll have to face going forward. Yeah. Well, I’m kind of happy the way we, we took this so far, I think we, we, especially in the US, we, and at some states, we air too much on the side of shutting everything down and telling people what to do, being very authoritarian, other states really opposite. But I think we came to a good solution, who knows if it’s going to hold, right? But it’s the way we also restructured our, our environment in terms of work that’s, that’s was coming for a long time. And some of it got stuck and hit this snack and it didn’t, it couldn’t, it had to snap, right? There was no other way to get out of this, this bind. And I’m glad it happened. And we will only be more productive. Hopefully we won’t all be lonely. That’s one of the downsides. People are really worried about that, the depression that comes on over. Even these things like these, these podcasts, like just, you know, these kinds of conversations, I think are, are great. And that’s, yeah, I mean, there are various apps that are trying to facilitate this kind of conversation and the serendipity of running into people that you never would have spoken with before, but you end up having some really pleasant conversations. And yeah, like it sits away for us to the bond and not just feel, you know, like lonely in whatever room we happen to be stuck in at the time. They say podcasts is the new coffee. Exactly. Yeah, I like it. I really enjoyed my coffee. That was awesome. Matej, thanks for coming on the podcast. That was really awesome. Thank you so much for having me. Yeah, I had a really great time. Thank you. Thank you for your insight. Thank you for your arguments. And I hope we get to do this again. Yeah, likewise. All right, looking forward to it. All right. Take it easy. Thanks a lot. Bye bye.